Sandra Peter and Kai Riemer
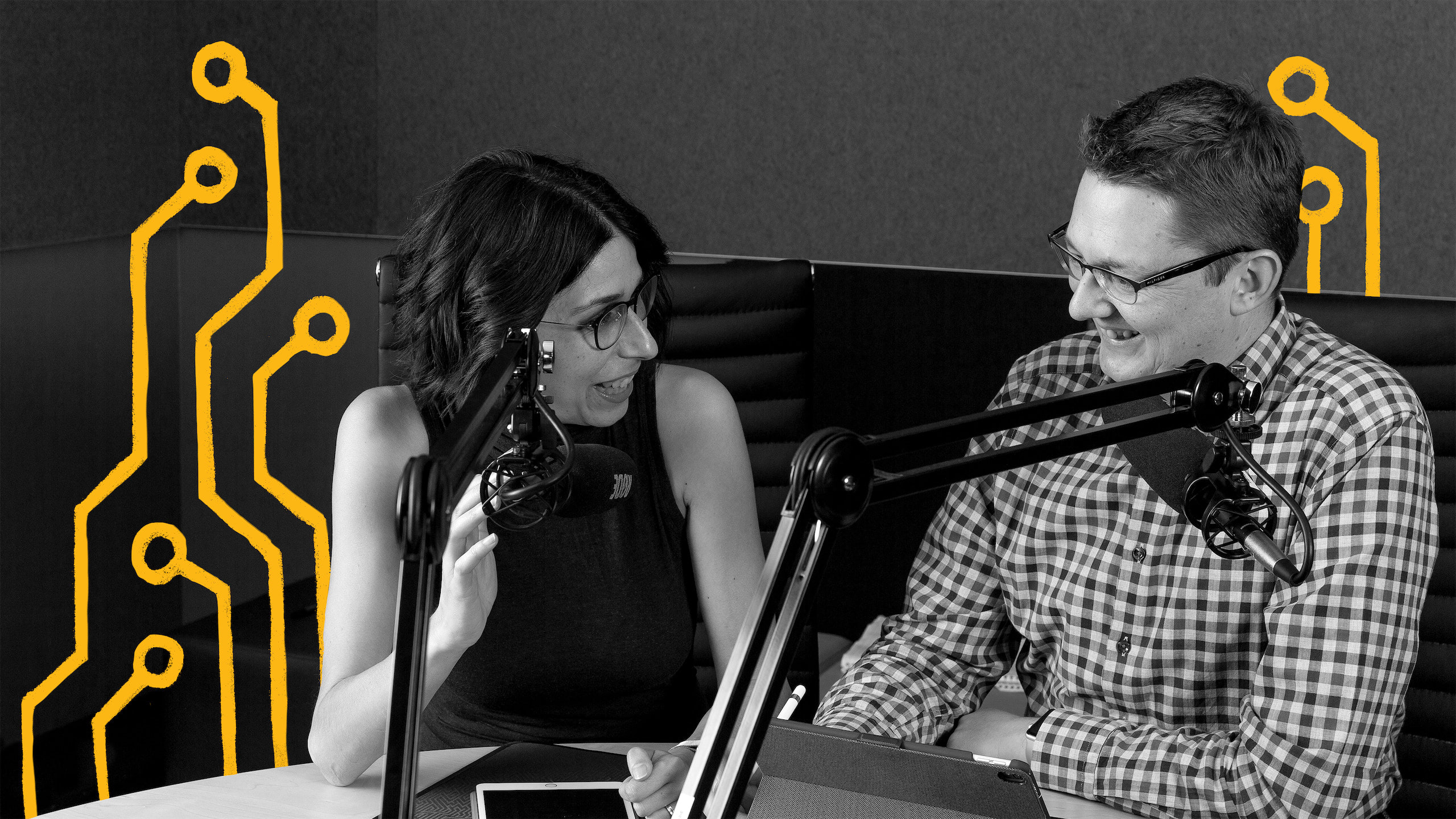
The Future, This Week 7 Dec 18: tasty app, fish faces, and car data
This week: food flavours, fish faces and China’s car data collection. Sandra Peter (Sydney Business Insights) and Kai Riemer (Digital Disruption Research Group) meet once a week to put their own spin on news that is impacting the future of business in The Future, This Week.
00:45 – Can an AI app map the way your food tastes?
13:36 – Facial recognition for fish
19:55 – Future bite: China’s data collection from electric cars
25:31 – Future bite: Google Duplex scripts now available for Pixel 3 phone users
The stories this week
The AI that can map the way food tastes (or can it?)
Other stories we bring up
Analytical Flavor Systems’s Gastrograph AI
What we really taste when we drink wine
CSIRO’s Wanda in training for fish recognition
The Nature Conservancy’s FishFace concept
Our previous discussion of Google’s Assistant and Google Duplex technology
Interview with Chinese official on electric vehicle data collection
Our previous episode on China’s electric buses
Future bites
In China, all electric vehicles send real-time data to the government
Google will pick up the phone for you
You can subscribe to this podcast on iTunes, Spotify, Soundcloud, Stitcher, Libsyn, YouTube or wherever you get your podcasts. You can follow us online on Flipboard, Twitter, or sbi.sydney.edu.au.
Our theme music was composed and played by Linsey Pollak.
Send us your news ideas to sbi@sydney.edu.au.
Dr Sandra Peter is the Director of Sydney Executive Plus at the University of Sydney Business School. Her research and practice focuses on engaging with the future in productive ways, and the impact of emerging technologies on business and society.
Kai Riemer is Professor of Information Technology and Organisation, and Director of Sydney Executive Plus at the University of Sydney Business School. Kai's research interest is in Disruptive Technologies, Enterprise Social Media, Virtual Work, Collaborative Technologies and the Philosophy of Technology.
Share
We believe in open and honest access to knowledge. We use a Creative Commons Attribution NoDerivatives licence for our articles and podcasts, so you can republish them for free, online or in print.
Transcript
Disclaimer: We'd like to advise that the following program contains real news, occasional philosophy and ideas that may offend some listeners.
Intro: This is The Future, This Week on Sydney Business Insights. I'm Sandra Peter. And I'm Kai Riemer. Every week we get together and look at the news of the week. We discuss technology, the future of business, the weird and the wonderful, and things that change the world. Okay let's start. Let's start!
Kai: Today on The Future, This Week: food flavours, fish faces and China's car data collection.
Sandra: I'm Sandra Peter, I'm the director of Sydney Business Insights.
Kai: I'm Kai Riemer, professor at the Business School and leader of the Digital Disruption Research Team.
Sandra: So Kai, what happened in the future this week?
Kai: Well AI is happening again. So our first story is from the Atlantic and it's titled "The AI that knows exactly what you want to eat.".
Sandra: Really?
Kai: No. And that's what we're going to talk about. Subtitled "Can an app lead to better tasting food by digitally measuring flavour?".
Sandra: So the article does recognise that flavour comes together through the way we taste things, to the way we smell things. And it's not something that you can easily analyse, easily grasp. Unlike things like sounds which you could measure with the microphone and you could have an objective measure for an objective level, the way we measure of flavour relies on the experiences that people have and hence have been fairly difficult to understand and comprehend.
Kai: So in that, sense taste and smell are probably the most subjective or inaccessible senses that we have. And we do not have a device that can capture the way in which we experience flavour in an easy measurement on a scale or in whatever form. As you say much like we could do with visual perception or audio perception. And so as far as a digital measurement goes, there's only the recourse via the human experience which then needs to be necessarily expressed in language.
Sandra: So the company Analytical Flavor Systems proposes a new app called Gastrograph.
Kai: A fairly unfortunate name, but never mind.
Sandra: Well it does aim to introduce a way to really reliably measure what we taste, reliably measure flavour. And that the hope is that as with everything else that we measure and we have data on, once we understand it, we can play around with it, we can control it and basically make the world a better place for everyone.
Kai: Not before we throw in a little bit of AI of course, which is the magic ingredient which will make all of this accessible. And so what the app does is, in the first instance, it allows people to express their flavour experience of a dish or type of food on a set of dimensions that is provided by the app. Presented visually in the form of a spider graph, where you can indicate on the set of scales. For example if the food is fruity, sugary, if it's wet, if there's spices present, if there's a floral taste. And then digging deeper into those different categories, you can describe what you are tasting in up to 600 dimensions. And so what the app actually does is it's translating a subjective experience into language categories. And the analysis that subsequently follows on the basis of this data is then a linguistic analysis. So presumably what happens is that the app collects a lot of these experiences, where people indicate what type of food they are tasting, which is the input data for the algorithm for the deep learning AI. What we call labelled data, which allows the algorithm to learn. And then as the article states, if someone is tasting something that they don't know what they are tasting, they can equally describe their experience in that way and the app would then be able to deduce what kind of food or dish they are actually tasting. So, so far, the understanding of what this thing does or what the article describes it does.
Sandra: So just make it clear, this is an app that is currently being used or tested by food companies but it's an app that anyone can download. And you can have your dessert and then enter your own data which the app will then compare to its entire body of data.
Kai: So it's a crowdsourcing approach. It relies on users to actually describe their experiences according to the categories and dimensions provided by the app. Which are language categories.
Sandra: So according to the CEO of Analytical Flavor Systems, the AI can determine the flavours in the food even better than you, the person who has submitted the food review. And even more than that, it can notice flavours that you do not consciously perceive and that can be so important for taste experience.
Kai: And so he goes on to say the app is literally reading someone's mind. But then quickly corrects himself.
Sandra: Oh thank God.
Kai: Yeah, he corrects himself. And he says, "No, if we were reading their mind they would've known they were tasting it. We're reading their subconscious."
Sandra: And this is the point where we probably need to start calling bullshit.
Kai: Yes. So no, there is no mind reading going on. There is no reading of the subconscious going on. Which is where it pays off to think a little bit more deeply about what this type of analysis actually does.
Sandra: So the app actually uses techniques from computational linguistics. What language researchers have tried to do previously is having large amounts of data, trying to find ways in which to group certain words, or certain parts of texts, or certain descriptions, and tried to create models of meanings. How do certain words relate to other types of words? Additionally, the app can do operations with some of these words. And the example that's given in the article is operations such as if you have the word 'king', minus the word 'man', plus the word 'woman', it gives you the word queen.
Kai: Which is a fairly simplistic understanding of language to begin with.
Sandra: And also of flavour. If you have a cracker remove salt, add sugar.
Kai: Yeah, gives you what?
Sandra: Weetbix.
Kai: Right. So the assumption that this app makes is that we can express flavour in language, so that there is a link between our experience of flavour and the way we express it in language. And that we can then make computation analysis, so reconfigure language and then...
Sandra: Infer something about flavour.
Kai: Exactly. Now let's look into this, because there's multiple problems. First of all, the makers of the app state as a problem of why the app is needed in the first place that people cannot explicitly pinpoint what they are tasting if they don't already know what they're eating. So they often don't have explicit knowledge of taste as such, of flavours.
Sandra: And the example here would be if you add the little bit of vanilla to a glass of milk, you will taste that it is sweeter and you would describe it as sweeter, but you wouldn't be necessarily able to pinpoint that it's actually vanilla that you're tasting in the drink.
Kai: Yeah. But at the same time they also claim that taste and the experience of taste is very subjective. Now at the same time they seem to say that the subjective expression of taste experience when aggregated like all our experiences, somehow lands us in a world where we have an objective description of taste that we can then manipulate on the language level to make inferences back to our subjective experience of taste. And that to me, right, while it sounds like magic is just bullshit because of first off, all the complexity that we're dealing with. Think of all the various ingredients and food types and dishes and recipes and all of the kind of things that we could possibly eat that would serve as labels for the data. And then the way in which taste is actually very contextual. It depends on what you have just eaten before. We know that hormone levels change the way in which we experience taste and temperature.
Sandra: And we know contextual cues influence the way you experience food. There are a number of famous studies that look at our experience of wine. The wine tasted better if it's poured to you from a bottle that has an expensive label on it versus a bottle that has a generic shop label on it. And these experiments have been consistently replicated in which the way the food is packaged, or are arranged on a plate, or served in a specific setting actually influences your subjective appreciation of how good that food or how good that drink actually is.
Kai: On top of that, we know that some people do have more precise tastebuds. They're better able to recognise and describe nuances in food. And on top of all of this, we also have the problem that people use language very differently, not only culturally but across age groups, across socio milieus. So not only do we have the sheer complexity of flavours and food types, varying abilities in the way in which people can taste and distinguish food, the contextual nature of flavour experience. We also have the variety in which we use language.
Sandra: And we know from our previous analysis of AI being used in different contexts, that the types of machine learning algorithms that we are developing now actually thrive in fairly bounded contexts. So we've looked at the learning algorithms that learn to play a game, or learn to recognise patterns in images, or learn to recognise faces. All of them require some sort of bounded context in which to make those inferences.
Kai: And good data. And this is where the problem lies. Because this app tries to solve the problem of subjective flavour experience by way of expressing subjective flavour experience in language. Which does not create the kind of data that you could use to tackle this problem, if indeed subjective flavour experience is a problem to begin with. Or is it not, maybe, what makes food and flavour so interesting? That we can have all of these varying experiences. So let's take a look at why this app exists and where this type of analysis might actually make some sense.
Sandra: And here we want to say something in favour of Gastrograph, because there are actually good places where you could use something like this. For instance, one of the examples in the article refers to Gastrograph being a good way for food manufacturers to get a better understanding of the consistency of the product that they produce. So imagine being a beer manufacturer or a coffee manufacturer where the quality of your product depends upon a certain process, but where you would want to ensure a certain consistency in the flavour that you're producing even though there are seasonal variations in the ingredients that go into your product. And here you would have tasters tasting let's say the beer or the coffee every season, and try to ensure that what you're getting out is a consistent product throughout the year or throughout the seasons.
Kai: So if you are drawing a sharp boundary around the number of food groups or flavours that are in play, and you are using a test group of people who use the same kind of vocabulary and language, an app like that could certainly work to explore how new combinations of ingredients or new flavour combinations might lead to certain experiences without actually having to produce those products to begin with. So it might be a way to simulate the way in which ingredients could affect taste.
Sandra: And indeed some of the customers the company has at the moment are just not only looking to maintain the flavour of their product, but also to figure out how to make incremental changes to the product lines that they have now to improve their product or to find new markets.
Kai: So I find this interesting right. It's a wicked problem, and I think it's worthwhile exploring to what extent we can express flavour in language and what we can do with this type of analysis. But I just want to point out that some of the claims that the article makes, such as predicting people's subconscious preferences. Or poking around in the thoughts that are secret even from ourselves in order to manipulate or hijack our mental processes, to entice us into eating certain foods. You know, is certainly not on the cards. So once again, here's an article which starts with an interesting problem, goes to an AI analysis which employs the kind of techniques that we've previously discussed, and ends up with claims that vastly overstate what these technologies can possibly do.
Sandra: But let's move on to our next article which actually does live up to the claims that it has around artificial intelligence.
Kai: So absolutely in stark contrast to this article, here is an application of this technology where it matters and where it actually works.
Sandra: And I absolutely love this article. It comes from Computerworld and it's titled "The Northern Territory Government using artificial intelligence to monitor fish stocks while avoiding crocs."
Kai: So we're talking the, what we call the 'top end' of Australia. One of the most beautiful parts of the world, with some of the most interesting water landscapes in the world. Beaches, but also rivers, with just a little catch.
Sandra: Yes, maybe not so little. So you've got sharks, you've got crocs. Which is why the Department of Primary Industry and Resources, together with - of all people - Microsoft launched facial recognition for fish.
Kai: So the problem being that if you're a scientist and you are researching the ecology of the ocean off the northern end of Australia. Or indeed the invasion of foreign species into the rivers of the national parks around Darwin, you do not necessarily want to put on your suit and dive because: sharks and crocs.
Sandra: Because: sharks, crocs and jellyfish.
Kai: Exactly. So what you'd rather do is you submerge a camera, you record video, and then you go and categorized and count the fish that swim past your lens.
Sandra: So your two options if you are trying to monitor fish stocks are either, as the article puts it, potentially deadly. Go down and count the fish, and you know - face the sharks and the crocs and the jellyfish. Or are deadeningly dull, as the article puts it. In which case you have people who have a PhD, sitting in front of a computer screen and basically going "fish, not fish, fish, not fish, fish, fish, fish".
Kai: For hours, days, weeks. Now, if only there was a technology that could help with that kind of problem.
Sandra: And this is where artificial intelligence comes in. And the Department partnered with Microsoft to try to develop a machine learning algorithm that would be an alternative to their "fish, no fish" problem. The solution that they developed, based on hundreds and thousands of hours of footage that this engine was fed, was that the AI system is now able to identify a fish in a video with 95 to 99 percent accuracy. And that's really amazing considering that the waters in many of these areas are very green and murky. There are huge tides, there is very low visibility. And the fact that the fish do not sit like in a passport photo, face the camera and then turned to the side and then turn to the other side, but rather swim around in a fairly disorganised and haphazard manner. So the algorithm has to actually be able to identify fish from every single angle, every position in different conditions of lighting. Something that is really not that easy a task to do.
Kai: And of course, you do not just want "fish, no fish", you want to tell apart Nemo from Dory. You want to tell apart different species, and then count them because that's the whole point of the exercise. So the way you do this is you have scientists trained the algorithm by doing the “fish, no fish" exercise for a while, and then you're in a position to actually automate that.
Sandra: So face recognition for fish.
Kai: So why should fish have it any better than us, right? Being under constant surveillance from CCTV cameras in public spaces.
Sandra: And this is of course not just the fight for equality for fish. Remember a while back we talked about the GoGo Chicken program in China that was doing facial recognition for chickens. There are also programs looking at koalas and sharks and so on. And Microsoft is not the only company doing this. So before we move forward, we must mention that there is similar work being undertaken by scientists from CSIRO, and Data 61 and of course the FishFace concept which won the popular vote for Google's Australia impact challenge a couple of years ago that was using facial recognition technology to look for a number of species of fish at sea. But the one thing we want to mention about this project that Microsoft has going on is that surprisingly the entire solution is available open source on GitHub. So this is something that can be used anywhere and by anyone and be adapted for any specific needs. And in this article the author mentions one such application which could be to actually do on the fly identification off catches on trawlers, on commercial fishing vessels. Currently those sorts of things are being done simply by weight when the fishing vessel comes back to port. Using this sort of technology you could do it as the fish is being caught, and looking at the specific species of fish that are being caught rather than just analysing things by weight.
Kai: And the issue here is of course reducing the number of by-catch fish that shouldn't end up in the nets, which can be minimised by picking the area in which fishing is done. And so you could mandate the installation of such cameras and algorithms on fishing trawlers in order to monitor and regulate better the way in which fishing is done to reduce the impact on the environment.
Sandra: And this of course comes at a time where discussions about fishery depletion and fisheries collapse is very much at the forefront of global conversations. And especially in Australia, there are warnings that the Australian commercial fish population could drop by a third in over 10 years. So finding these sorts of solutions and applications for AI is actually very, very timely.
Kai: Or you could of course just install this technology in your home aquarium to keep taps on your own little fish population at home.
Sandra: Okay so it's time for short stories.
Kai: Future bites.
Sandra: My future bite comes from AXIOS, and the article is titled "Electric vehicles in China send real time data to government." And what the article mentions is that there are more than 200 manufacturer selling electric vehicles - and this includes big names like Tesla and BMW, and Ford, Daimler, Nissan, Mitsubishi - who transmit information, things like location. Or who transmit the number of data, things like the location of the vehicle, and speed and so on, to Chinese government back monitoring centres. And this is, the article reports, quite often than without the knowledge of the car owners. And let's make it clear that these car manufacturers are actually complying with local legislation, local laws, which apply to all alternative energy vehicles.
Kai: So this is really interesting. There are currently more than 220,000 vehicles on the road. And all of this data, geo location, speed but also engine telemetries and data are about the car itself, the battery the charging levels, and all of this is transmitted and then collated in real time in what is called the Shanghai Electric Vehicle Public Data Collection Monitoring and Research Centre.
Sandra: That's a mouthful.
Kai: Yes. And they have a real time map where they can see where all electric cars are, and what their charging levels are, and can then use this for what?
Sandra: What most articles reporting on this news have highlighted was the surveillance angles. And everybody said that well, this just adds to the rich numbers of ways in which the Chinese government surveils its population.
Kai: And you can't blame the media, given all the recent news about facial recognition in public spaces, and the way in which China does indeed use this data to keep tabs on their population and to identify criminals, apprehend people that are wanted. But there are also a couple of articles which report on an interview with Ding Xiaohua, who's the deputy director of the Shanghai Centre, who says that the centre is actually not designed to facilitate state surveillance. Though he admits that the data can be shared with police or prosecutors’ courts if a formal request is made. And he says quite facetiously that state authorities have many more other ways to actually monitor people, and this is also only a very small subset of the population. So what is this data likely good for?
Sandra: Where the interesting value of this data lies is actually in the ability of local governments or national governments to use that data to better improve, for instance, city planning. If you're looking to use that kind of data for instance, to optimise traffic. If you're using that kind of data to actually build a network for electric vehicles and to improve the adoption of electric vehicles to try to supplement other types of information that you might have about how people move around, how they use private versus public transport, you can view the types of data that they are obtaining from these electric vehicles in a whole new light.
Kai: And let's not forget that China has plans to electrify most of its fleet in the coming years. They're making a very big push in light of climate change and pollution in cities.
Sandra: And we spoke previously about the electric buses in China, which have more than pretty much any other place in the world. We'll include the link in the shownotes.
Kai: So as these cars come online in China, they have the ability very early on to plan the location of charging stations, to learn more about the usage of these cars. And therefore to plan in a fairly top down way the infrastructure and rollout of this technology in ways that are inaccessible to authorities in the West arguably.
Sandra: And there is also a second dimension to this type of data in China. Which is the fact that this kind of large-scale data can be used to train better AI, better machine learning algorithms. Where the Chinese have an advantage compared to places like the European Union or the US or even Australia is in the fact that the government collects these data and then also shares this detail with both state owned and private organisation in the push to rapidly improve the AI that they develop. Whilst in the US or in the European Union there are increasing barriers set up to either protect the privacy of citizens and their data, or barriers that private companies set up to protect the data they collect. In China there is much more significant effort to leverage the data that the government collects in private-public collaborations.
Kai: And finally, here's an angle which I haven't seen in any of the articles on the topic. And that is that the Chinese authorities collect all of this data from cars from, all kinds of manufacturers chiefly premium Western brands such as Tesla, Volkswagen, BMW, Daimler and so forth, in order to learn about the performance of electric vehicles. And who is to say that this data is not being shared with the state-owned car manufacturers who are ramping up their production and development of their own electric cars.
Sandra: So definitely something to keep an eye on. But we have time for one last few tonight. What's your short story of the week?
Kai: Very short, literally because this is just a note from Engadget. Google's call screening transcripts roll out to Pixel owners. So those owners of a Google Pixel 3 smartphone now have access to Google assistant, who can screen incoming calls where the caller is connected to the Google assistant AI synthetic voice.
Sandra: And by synthetic voice we mean the Google Duplex assistant that we featured previously on the podcast. Here's a little clip to remind you of what that sounds like.
Google Assistant (file audio): I'm calling to book a woman's haircut for a client. Um, I'm looking for something on May 3rd.
Sandra: As you can see, it's virtually indistinguishable from a human voice. And they will now be your private assistant.
Kai: And so the caller has to put up with this artificial voice, leave a message and then this message and the interaction is accessible as a written transcript and the owner of the phone can then decide whether or not to follow up with the caller.
Sandra: So Megan, if Kai calls, we can basically let the assistant pick up. Oh hang on - neither of us have a Google Pixel phone. But that's ok. We could get one, and then decide whether we want to return Kai's call or not.
Kai: Only time will tell how comfortable or annoyed indeed people will be when they are frequently talking to, you know, artificial Google rather than real Sandra.
Sandra: But how would you even know if it spoke with my voice?
Kai: That's all we have time for today.
Sandra: Thanks for listening.
Kai: Thanks for listening.
Outro: This was The Future, This Week made possible by the Sydney Business Insights Team and members of the Digital Disruption Research Group. And every week right here with us our sound editor Megan Wedge who makes us sound good and keeps us honest. Our theme music is composed and played live from a set of garden hoses by Linsey Pollak.
You can subscribe to this podcast on iTunes, Stitcher, Spotify, YouTube, SoundCloud or wherever you get your podcasts. You can follow us online on Flipboard, Twitter or sbi.sydney.edu.au. If you have any news that you want us to discuss, please send them to sbi@sydney.edu.au.
Kai: So why should fish it ship. So why should fish. So why should fish - ah! So... yes
Sandra: Try again.
Kai: Face. Face recognition of facial fish. Fish. Fish. and.
Sandra: Facial recognition for fish.
Kai: Fish. Fish.
Sandra: Fish faces.
Kai: Can you say fish. Fish facial. Fish facial recognition. Fish face.
Close transcript