Sydney Business Insights
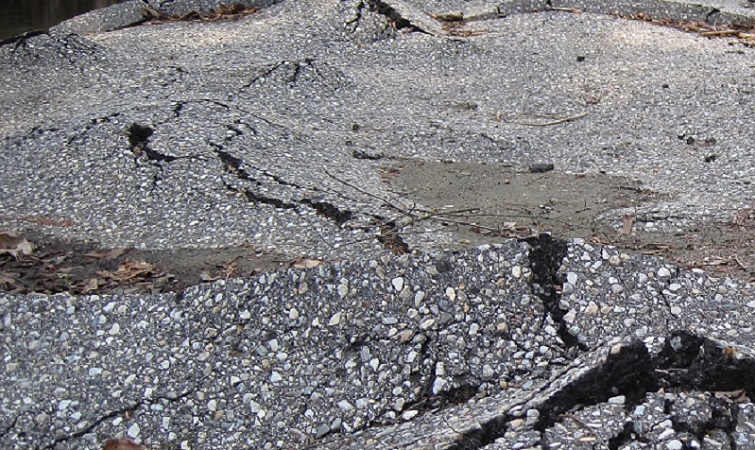
A bumpy road ahead
The culprit in the recent fatal autonomous vehicle incident may never be identified because the truth is, it may not be technically possible to determine what informed Uber’s AI algorithm that was driving the car to act in such a way causing it to hit Elaine Herzberg.
If it turns out that no other component (such as a sensor) is to blame, then surely it’s time to ask ourselves – is it really OK to hand over the car keys to an AI ‘blackbox’ and, what sort of driving future do we want?
Of course the search for mechanical answers must be pursued (more on this below).
But given the possibility we may arrive at a mechanical dead-end, we must also examine the reasons for introducing such vehicles. Why do we want them? What service will they perform that is not already capably carried out by other transport modes (like, public transport)?
And fundamentally we need to be squaring up to the issue of whether the compromises needed to get autonomous vehicles up and running are worth it – because for sure AVs are not feasible under current urban conditions. So what would we be prepared to change: Limits on where people can walk? Taking cyclists off roads? Building AV-only lanes?
But first let’s understand the technological unknowables driving autonomous vehicles.
Listen to Sandra and Kai discuss driverless cars on The Future This Week
We are indebted to Martyn Thomas, a professor of information technology at Gresham College who explained how Uber and its competitors trial self-driving cars, and compares this with how engineers evaluate new technology. In a scientific experiment you have a hypothesis that you are trying to prove. The point of a test is to establish some form of causality: you have a certain expectation of what ought to happen when you put a technology to use, and then you compare that with what actually happens. If there is a deviation, you deal with whatever caused the deviation.
But, as Professor Thomas explains, Uber et al are “conducting a set of random experiments with no structure around what exactly they need to achieve and why these experiments would deliver exactly what they need to achieve.”
The Uber autonomous vehicle involved in this crash was using a combination of material technologies, things like sensors, video, radar and lidar (distance measuring lasers). These technologies allow the steering unit of the car to identify objects and also measure the distance to those objects, predicting where they are in space and in time.
The data collected via the sensors is fed into a set of self-learning algorithms that make up the steering unit. This unit processes that data and adjusts the car controls accordingly.
We know the car involved failed to break before hitting Ms Herzberg. We don’t know yet if it was an error in the sensors (misreading the scene) or because the algorithm processed the data incorrectly – or, a third possibility, if this truly was an accident that could not have been prevented.
But whilst the investigation is afoot, Professor Thomas points out that with self-learning algorithms we are not in a position to create conditions under which scientific testing is normally done: That’s because as these algorithms constantly learn they change all the time: so as the cars are being driven around testing them in different situations, the configuration that is being tested is constantly changing. Also the systems’ hardware, software, the technology being deployed, is being updated and changed by the company all the time. Which raises the question – how are Uber and other AV companies actually able to test, and therefore improve, the technology in a truly systematic and reliable way?
Ironically – it’s in this constant improvement that the problem lies. Are they improving?
What these algorithms are really doing is adjusting the internal weights in the network of statistical values, coming to ever-changing adjustments to the outcomes produced. And while we typically frame such changes and adjustments as ‘learning’, technically any change could be introducing new problems also. So who is to say that as the network is ‘learning’ that certain improvements in one aspect of behaviour of the car might not override (therefore technically forget) other behaviours, so it’s never clear how a car will react in a certain situation? As far as ‘scientifically testing’ autonomous vehicles goes, it is impossible to know if you are on a pathway to truly improving the behaviour of the car across all possible situations.
If an accident doesn’t occur we have no way of telling whether the algorithm got better or worse at what it was learning: Maybe it learned to observe less things in its environment because it had no accidents and therefore did not have the opportunity to correct itself. There may be situations it still cannot anticipate but those remain buried in the algorithm and we have no way of telling if the car has improved or not.
In the wake of this sad case a host of self-interested parties – technology providers, other AV producers – have bobbed up stating the incident has nothing to do with their tech. Predictions about the causation and therefore blame are all lively issues but the overarching argument fueling the agenda is that there will be fewer road accidents and therefore death/injuries, in a streetscape dominated by autonomous vehicles. But, life is messy, accidents will happen – any system involving humans is never going to function totally reliably.
So if we accept an AV future as inevitable – Ford and BMW are both promising consumers autonomous vehicles by 2021, do we need to set up an environment where humans and AVs interact as little as possible?
Just think about a regular urban hot spot: Lots of pedestrians crossing all over the place, cyclists zipping in and out, children playing, and coffee shops with outdoor seating. Would that social and community environment be made better if there were less accidents, less fatalities but if all pedestrians would have to keep to specific paths, if you don’t allow cyclist to go on the road, if the entire community was reorganized to accommodate these autonomous vehicles?
At what cost do we actually want to introduce self-driving vehicles into our environments and what problems are they actually solving? If it is just to have a safe commute from A to B, we’ve already solved this problem: it’s called public transport. We can have fully automated trains, for example, that are pretty good and do not cause many fatalities.
And if the true reason for the existence of cars is the freedom to go …anywhere – well can autonomous vehicles offer that same flexibility?
So rather than only having blanket conversations about how we test and regulate autonomous vehicles it is also important to have conversations about why we want them and where we want them. And 2021 is getting very close.
You can subscribe to this podcast on iTunes, Spotify, Soundcloud, Stitcher, Libsyn or wherever you get your podcasts. You can follow us online on Flipboard, Twitter, or sbi.sydney.edu.au.
Sydney Business Insights is a University of Sydney Business School initiative aiming to provide the business community and public, including our students, alumni and partners with a deeper understanding of major issues and trends around the future of business.
Share
We believe in open and honest access to knowledge. We use a Creative Commons Attribution NoDerivatives licence for our articles and podcasts, so you can republish them for free, online or in print.