Jevin West, Sandra Peter and Kai Riemer
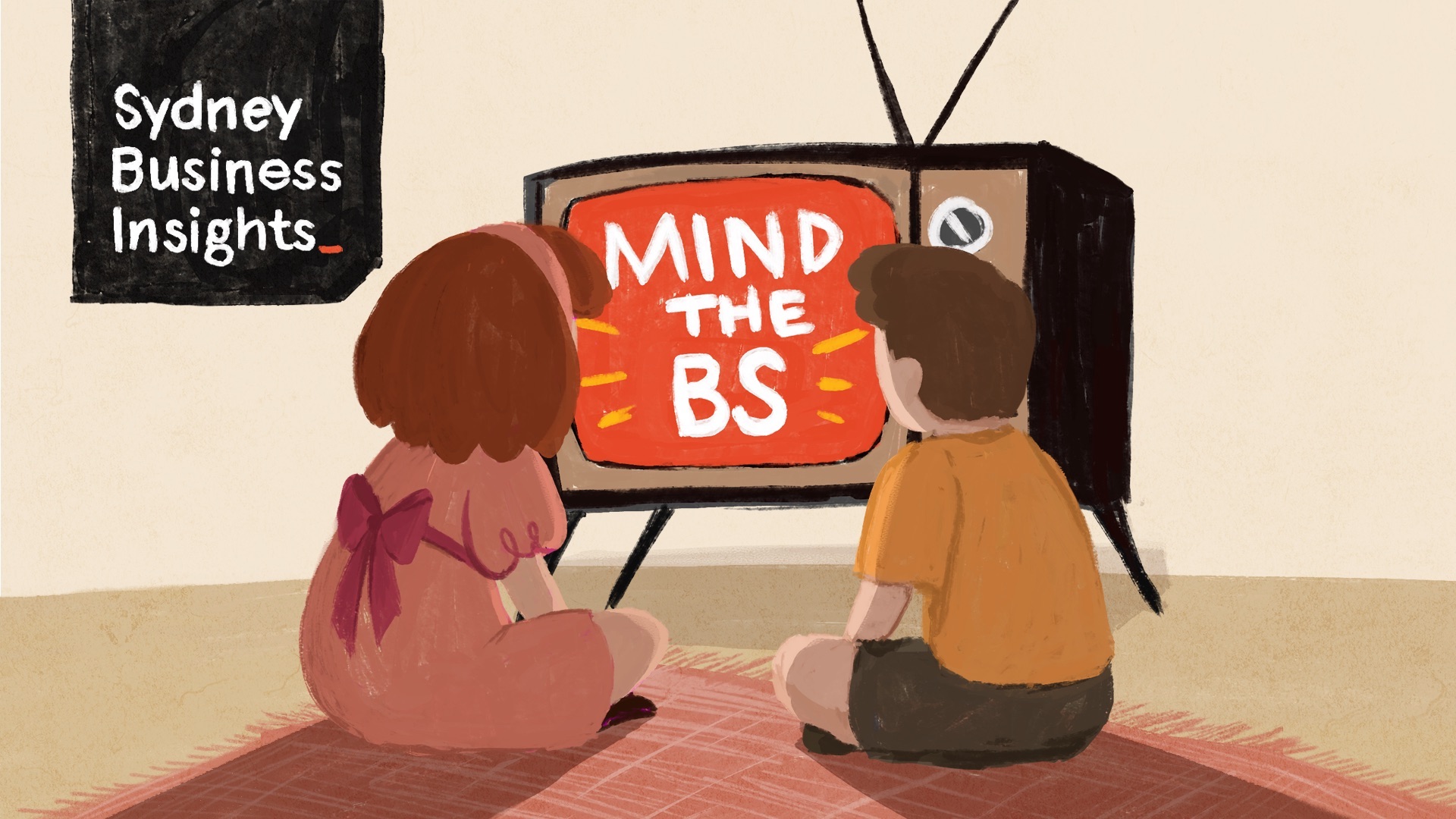
Calling bullshit and algorithms on The Future, This Week
This week: calling bullshit, sexist credit cards and death predictions with Jevin West. Sandra Peter (Sydney Business Insights) and Kai Riemer (Digital Disruption Research Group) meet once a week to put their own spin on news that is impacting the future of business in The Future, This Week.
Our guest this week: Associate Professor Jevin West
The stories this week
00:45 Toilets are dangerous business, World Toilet Day
06:02 Apple’s credit card is being investigated for discriminating against women
19:41 AI can tell if you’re going to die soon, we just don’t know how it knows
Other stories we bring up
Calling Bullshit: data reasoning in a digital world
Jevin’s research centre, The Centre for an Informed Public
Jevin’s subject: Calling bullshit in the age of Big Data
Jevin’s TedX talk: We’re drowning in BS, but you can learn how to fight back
Jevin’s talk at CreativeMornings Seattle on bullshit
Japanese high-tech toilets are conquering the west
Software developer David Heinemeier Hansson complains on Twitter
We teach A.I. systems everything, including our biases
Our previous conversations about the ethics of AI
Our discussion with Professor Daniel Kahneman on bias and algorithms
Our previous conversation on women and the future of work
You can subscribe to this podcast on iTunes, Spotify, Soundcloud, Stitcher, Libsyn, YouTube or wherever you get your podcasts. You can follow us online on Flipboard, Twitter, or sbi.sydney.edu.au.
Our theme music was composed and played by Linsey Pollak.
Send us your news ideas to sbi@sydney.edu.au.
Jevin is an Associate Professor in the Information School at the University of Washington and Director of the Center for an Informed Public.
Dr Sandra Peter is the Director of Sydney Executive Plus at the University of Sydney Business School. Her research and practice focuses on engaging with the future in productive ways, and the impact of emerging technologies on business and society.
Kai Riemer is Professor of Information Technology and Organisation, and Director of Sydney Executive Plus at the University of Sydney Business School. Kai's research interest is in Disruptive Technologies, Enterprise Social Media, Virtual Work, Collaborative Technologies and the Philosophy of Technology.
Share
We believe in open and honest access to knowledge. We use a Creative Commons Attribution NoDerivatives licence for our articles and podcasts, so you can republish them for free, online or in print.
Transcript
This transcript is the product of an artificial intelligence - human collaboration. Any mistakes are the human's fault. (Just saying. Accurately yours, AI)
Disclaimer We'd like to advise that the following program may contain real news, occasional philosophy and ideas that may offend some listeners.
Intro This is The Future, This Week on Sydney Business Insights. I'm Sandra Peter, and I'm Kai Riemer. Every week we get together and look at the news of the week. We discuss technology, the future of business, the weird and the wonderful and things that change the world. Okay, let's start. Let's start!
Kai Today on The Future, This Week: calling bullshit, sexist credit cards, and death predictions with Jevin West.
Sandra I'm Sandra Peter. I'm the Director of Sydney Business Insights.
Kai I'm Kai Riemer, professor at the Business School, and leader of the Digital Disruption Research Group.
Sandra So, Kai, what happened in the future this week?
Kai Well, we have Jevin West.
Jevin My name is Jevin West. I'm an Associate Professor in the Information School at the University of Washington in Seattle. And I co-direct the DataLab, and now direct a new Center for an Informed Public. And my area of expertise is bullshit studies.
Kai Hello, Jevin. You'll fit right in.
Jevin Thanks for having me. This is like a culmination of my career so far.
Sandra And we've brought you in for what is soon to be World Toilet Day.
Jevin And what a perfect time for me to ask your audience if they could answer the following question, which I have asked to my class for real, I'm not bullshitting you here. That, according to one viral meme that passed around the Internet not too long ago, 30000 Americans are injured each year on the toilet. True or false? I should look to you, Sandra and Kai. True or false?
Sandra Oh, this is a tough one. 30000 Americans. That sounds like a lot.
Kai Well, there's a lot of Americans too, right?
Sandra About 350 million of them.
Kai Yeah. So 30000 is not a lot.
Sandra A year, is it?
Jevin Per year, yes. Good question.
Kai So what do they do? Like they get injured, like, hit their head on the toilet lid on their way up, or?
Sandra On the way up! Or do they injure themselves on the way down?
Jevin I, I think both directions are, are categorised.
Kai Working too hard, cardiac arrest?
Jevin Yeah. There's something that people call the valsalva manoeuvre.
Kai Oh, fancy word.
Jevin That's one form of injury. Yeah, so you guys are asking the right questions.
Kai It's true, isn't it?
Jevin It is true.
Sandra Not Bullshit.
Jevin This one's not bullshit.
Kai And these are reported cases, right. So the dark number is probably much higher.
Jevin That's right, the dark number of people not reporting, it's gonna be much higher. But you guys did the right thing, it's something we call 'fermi estimation'. This is this ability to ask questions in sort of orders of magnitude, and getting a pretty good answer, but it turns out this one is true.
Sandra In all fairness, it is going to be World Toilet Day soon, which is the 19th of November and it is the United Nations international observance day to inspire action to tackle the global sanitation crisis. And this is another stat that's not bullshit, that 4.2 Billion people live without safely managed sanitation and more than half a billion people, that's almost 800 million people practice open defecation. These are terrible statistics on World Toilet Day.
Jevin So that's over half of the world, basically.
Kai That is terrible. And obviously, people are working on this, among them, Bill Gates. And there was an article recently that I saw which basically took Bill Gates to court about the way in which this is being approached, because creating a Silicon Valley-designed $50000 toilet is not scaling to solve a problem of this magnitude. So these are pernicious problems that really need large-scale social change more than a new gadget.
Sandra Yep, but do get involved, worldtoiletday.info.
Kai Yeah. After two weeks ago, we gave you a hashtag, #GiveAShit for science, this week...
Jevin #GiveAShit for toilets.
Kai Absolutely. And just to cross-reference and give a shout out to, you know, one of the other great podcasts out there. WIRED UK, just a little while back, had a great episode about Japanese high tech toilets. So that's a good one to listen to.
Jevin You know, this is a show about the future. And if you go to Silicon Valley, those discussions are all about flying to Mars, or coming up with some new device that's going to hook right into your brain, when in fact there are people dying because we can't put enough toilets in the world to serve them. So maybe this, when we talk about the future, maybe the future, there's a lot more places to use the bathroom, I think. And that's something we should start focussing some of our tech Silicon Valley over idealism towards just as much.
Kai Jevin, we have this Juicero award on the podcast, which we haven't awarded in a while. Juicero wants this $700 juice-bag squeezing device, completely over-engineered.
Sandra Turns out you could do exactly the same thing with your hand.
Kai And so the high tech toilet designed with all the design thinking and the innovation methods that Silicon Valley has to offer...
Sandra That would of course require a new sanitation system and everything else would have to change, not just the toilet.
Kai Absolutely, yes. And it is all well and good. But some of those problems will not be solved by developing gadgets, right. So we need to be mindful of that, that sometimes when we talk tech, we're talking to one part of the world and not to another.
Jevin You know, one of my favourite gadgets is the app to remind me to drink water, or the new device that is the $200 salt shaker.
Sandra Tell me it's not true.
Jevin This is the kind of thing that we're inventing instead of solving big things like getting toilets and all the other aspects that go into building a proper sanitation system,.
Sandra Which, by the way, would advantage women a lot more. Young girls and women seem to be most disadvantaged by not having a toilet.
Kai They also seem to be disadvantaged by Apple's credit card, by the way. Which brings us to some of the topics for today. So what are we talking about this week?
Sandra So this week we've got Jevin here, so we do have to talk about bullshit and artificial intelligence and machine learning.
Jevin I'm glad you put those all in the same sentence because they tend to fall in the same sentence a lot.
Sandra So our first story comes from The Verge, and it's titled: "Apple's Credit Card Is Being Investigated for Discriminating Against Women". So this whole thing began when a software developer complained on Twitter. Quite a prominent software developer, with about 35 thousand people following him, wondered how his credit line could be 20 times higher than his wife, and referred to Apple's credit card as a sexist program with a number of expletives added there for emphasis. So him and his wife would file joint tax returns. And he was the one who had the worst credit score, yet the credit that was offered to him was 20 times higher than the one offered to his wife. So. Bullshit or not, Jevin?
Jevin I think this is another example where the algorithms on their own can make these kinds of mistakes, and because they're very, very hard to reverse engineer, you know, we depend on these anecdotes. I mean, they are anecdotes. But this kind of thing keeps rearing its head, from the ways that Uber treats women and men differently, to the ways in which hiring is separated using algorithms. I mean, Amazon's attempt at disrupting another industry through automatic hiring and of course, removing all gender bias did not come to fruition because when you use resumes, you can't just take out the names. There are all sorts of other features and data inside that make the biases really difficult, if not impossible to remove.
Kai So there was an interesting statement from Goldman Sachs, which is, of course, the financial provider behind the Apple Card. So, you know, Apple provides the tech and the payment system, but the actual credit card is underwritten by Goldman Sachs. And they came out and said, in all cases, we have not and will not make decisions based on factors like gender. And I thought this is a fairly confident claim to make given that the algorithms that are being used in these systems these days are fairly opaque. And so they work on this data, and apparently, you know, according to a few stories that we've heard, they do make quite gendered decisions and they have gendered outcomes. So to say that they don't, how can they make that claim when they don't even know how the algorithms make those decisions?
Jevin I think it saves them legally by just saying they don't. But even if you look at their current hiring practices or for any sort of big company like Amazon, for example, they would probably claim during their hiring over the last 10 years they don't have discriminatory practices in hiring. But if you look at their current workforce, or any and it's not just picking on Amazon, it's any of the industries that have shown retrospectively that there is gender bias, then yes, making that claim is vapour claiming.
Sandra So let's unpack this. How does this actually work?
Jevin Well, anytime you're talking about machine learning, generally, you're talking about a process that requires data as the food for these algorithms. And when that happens, you're using data that already exists. So the case of Amazon, they were training on existing resumes, and that trained the algorithm to look for features that would lead to successful employees. Now, the engineers likely said, well, we can't look at gender, so let's pull the name out. But there are other features that correlate well with gender and those were likely not taken out. And so the problem is the machines don't just poof, come up with decisions they needed to be trained on data. And the data itself has these biases already in it.
Kai So if you're a current hiring practices are already privileging men over women, then you're just going to do this in the future, right?
Jevin Exactly. That happens over, and over and over again. And there's really no magical formula for getting around it. There are discussions right now in the research world about creating synthetic data, or creating data around worlds that we want. But that's not as easy as it may sound, and getting there is quite difficult. So the problem is that we need to at least recognise that this is going to happen, and when a company says, 'oh, we don't have bias issues, our algorithms and our engineering teams are gonna do this without bias', you can start to question them right away.
Kai And what do we say then?
Jevin Bullshit.
Kai Exactly.
Sandra And to be fair, this happens in all large companies. So in this case, it was a company like Goldman Sachs. You brought up Uber, you brought up Amazon, and these are large companies with very good data scientists, and arguably some of the best teams in the world having to look at this. And even they cannot remove the bias from the data that they already have, or think of ways in which we can sanitise data, whether that's for hiring purposes, or whether that would be for health care or for housing or anything else we wanted for.
Kai Let's bring up another one, because there's just now in The New York Times, an article this week that looks at Google, and the program that they have developed called Burt. Which is a linguistically based deep learning algorithm which has been trained on a large corpus of text from books and Wikipedia articles and online articles to make language-based predictions. We've discussed the open AI text generator just last week, so this is a similar one. Which basically generates new text or makes predictions about what the next word in a sentence should be, based on the texts that already exists. And Google is using this to dramatically improve the predictive nature of search results, for example. And you know, when you type into the search bar, it sort of gives you what you might likely want to type and makes suggestions. So in a sense, it works quite well. But it has been shown that, again, this data is heavily biased, it's gendered and there's a problem there. So how does that come about?
Jevin I mean, it comes about for the same reasons that we mentioned before, but it reminds me of related examples of this. So if you go into Google Translate, for example, and you translate 'doctor' or 'nurse' in one language, let's say Turkish, and then you take it back into English or whatever language you're trying, you can see immediately that the algorithm itself has this bias. It will say 'he, the doctor' or 'she, the nurse,' even though you gave it no gendered information. It also reminds me of some of the work that Sean Munson did, actually at my university, where they did something very simple. They said, 'let's ask Google Images, we'll just put in CEO'. And from that output, you can then count the number of women that are represented as the canonical CEO. Well, it turns out it's highly biased towards males. If you do that search right now, you find almost all males. But if you actually add up the Fortune 500 CEOs, there are a lot more women that are represented in any of the results in the studies that he has done. And so this also is another indicator of how it's just built in with the data. So these kinds of things rear its head all the time.
Kai Let me ask the provocative question then. Are the algorithms biased or accurate? Because the problem is not that we make them biased, the problem is that they reflect our current world, which has all kinds of inequalities in them. The algorithms, ironically, quite accurately reflect what the world is like right now, which is heavily gendered maybe, which has discrimination against minorities based on race, based on age. And so that gets reflected in the language that is used in books where, you know, typical jobs are associated with men or women. And so 'programmer' then is associated with the male pronoun in the algorithm, which is because most programmers in Silicon Valley, for example, are men, not women. So the problem to me seems not that there's this inequality baked into the algorithm, but what we're using the algorithm for, because we then utilise the algorithm to make predictions about or shape the future in some way, which we don't want to reflect those same inequalities.
Jevin So I think you're absolutely right. I don't want to downplay your provocative question. I would almost not make it provocative because I think it's right on. It comes from the need for us to separate the algorithms themselves. So whether you're talking about a random force algorithm, or a deep neural net, or whatever method you want to use as your algorithm from the data that's ingested. Now, there's multiple kinds of machine learning that goes on, there's the unsupervised and there's the supervised. But most of the kinds of things that we're seeing in the world depend on supervised machine learning, which requires this labelled data. So the way we think about it, when we're sort of calling bullshit on technology, or on statistics or algorithms, my colleague and I, Carl Bergstrom, we have a class and a book on this where we basically separate the black box. That's the algorithm. Before that is the input data, which is where we say 'focus your intention on the input data that had to have gone into that black box to make the decisions, the output data that comes out in the interpretation of that. Ignore the black box'. And when we talk about the black box, that's not what's biased or racist. The algorithm is crunching a reality of the world. And we need to be aware that that reality, that data, is needed by the algorithm to make predictions, but it's also making predictions that might further foster that bias.
Sandra So to clarify a few things for our listeners who might not know, you teach a class on calling bullshit. How did that come about?
Jevin Well, my colleague Carl Bergstrom, who's also a professor at the University of Washington, came about many years ago, far before, like, the 2016 election. Everyone thinks, 'oh, that was a response to the election'. We had been seeing so much bullshit in our professional lives, we work in science and research, and also in our personal lives. It was reaching a level we couldn't take anymore. And we thought the most important thing we can do for students and the public is to teach a class on how to not just spot bullshit, but how to refute it. It's different, because there is a whole set of lectures and efforts that we spend upon spotting bullshit, but also in the refutation. So that class is meant to sort of go from philosophy, to data science, to machine learning and talk about the ways in which we can detect and refute bullshit.
Sandra So you said 'ignore the black box, and look at the data that's coming in and what's coming out'. In this case, it looks quite easy to see because the algorithm was sexist, let's say, and you see the way in which it discriminates. Quite often we see discriminating against certain minorities, or age groups or other things that are quite visible in the data. What do we do about non-obvious biases where things are a little bit more subtle? How do we spot those? These are things we know to look for. But how would we approach an algorithm where it's maybe a little bit more subtle?
Jevin I think this is something that we need to be doing as a research community and in the tech industry, and I think there's some business opportunities. I mean, some are already talking about a multi-billion dollar industry auditing algorithms and things. So I think part of it would be having some standards that made at least parts of the algorithm more transparent. I mean, I think there is some real value and I think it's oversold about what transparency is because, sure, you can open up a big multi-level neural network, but good luck in trying to figure out how it's working. But still, we want to provide information at least where the data came for doing the training. We want to have at least someone on the other side of the black box going, 'Okay, you know, we've done some of these standard tests about where there might be biases. What are some other things we can look for?' So I think it's very important to go beyond what you're saying, the easy things to look for in biases, and to look for more subtle things that might have regional differences, for example.
Kai But I'd like to push you a little bit further then because, of course, we know sexism, ageism, racism. These are the obvious ones that we can look for. But there's all kinds of ways in which inequalities get reflected in the data that are present in our work, that we might not immediately pick up on. And because we don't know, if we feed like 40, 50, 60 different data points into the algorithm, we ultimately do not know which ones will discriminate in the end, when the algorithm passes judgment on a single future case. Are there certain areas where we shouldn't use these kinds of algorithms then? And it's obviously a rhetorical question, but I'd like your opinion on it, because if we cannot be sure how the algorithm propagates certain inequalities, then is it safe to use them?
Jevin I think this depends on the application. So if it has to do with people's health, if it has to do with whether they're going to be re-placed in jail, whether it has to do with a loan, large consequence algorithm, should receive more inspection and should have more of the spotlight on it. And we should be more reserved about just turning over an algorithm to predict whether you're going to need care in an emergency room or not. I mean, come on, at this point, humans still do a pretty darn good job and 12 years of medical training. I trust that before I trust some of these experimental methods. But that doesn't mean we should throw out all efforts to incorporate and augment, which might be a better term for artificial intelligence. This 'augmented intelligence' might be a better way of thinking about all this, that we spend more time thinking about how, you know, doctors could use them as another piece of information. I mean, although that could influence and there are these kinds of things to worry about as well. But I think for low-cost or low-impact events. Go ahead, let's try the machine. But for those things that matter. I think your point is well taken. We don't fully understand them enough that we at least have to have sort of a pseudo moratorium. Basically have conversations like we're having right now with experts in the field and figure out where we can reduce some of the risk.
Sandra You mentioned healthcare in the medical space, which brings us to the other story we wanted to discuss with you today, which is from Popular Mechanics: "AI Can Tell If You're Going to Die Soon. We Just Don't Know How It Knows". This was an article in which apparently the researchers found that a black box algorithm predicted patient death.
Jevin That's a great selling headline, "Predicting Death". It almost sounds like we need to put the date on a calendar now. I think that this idea of writing in your headline, the ability to predict death, is really almost as much the problem as the research claiming that it's done that. Now we could go into details about what they did, but this kind of thing generally happens all the time. And, you know, journalists have a hard job to do. They're trying to compete their headline against another headline. And so predicting death is something that, you know, some people refer to as clickbait, some refer to it as forward referencing. But this idea of being able to look at an EKG, an electrocardiogram, and then be able to predict who needs care or who's going to die if we don't. We're so far from it. This was a study that had some positive results, but we are so far from being able to predict death.
Kai Exactly.
Jevin No matter how deep your neural net is.
Kai And we don't even know the science properly, because this was done by a private company, it wasn't peer reviewed published research. And there's question marks over the methodology. And so one of the things we wanted to highlight with this is just sometimes the outrageous claims that are being propagated in the media, and which then contributes to the general public's view of artificial intelligence as these incredibly new thinking machines, making judgements better than humans. You know, it's almost akin to magic. And why wouldn't you use it in all these various areas when it's supposedly so much better than the fallible humans, and objective, and machines are accurate? And so a lot of the decisions being made are driven by the frankly bullshit claims that are being propagated in the media, which are not in the science.
Jevin Yeah, I think a better way to report this would be, 'Yeah, we improved predictions by 5 percent when compared to medical doctors over the same time period. But wait. Those medical doctors didn't have access to all 40000 EKGs. You know, in this case it was only one year time span. So it didn't take into account all the other context that would go into a decision a doctor would have...'.
Kai Yeah, boring though, right? That doesn't sell.
Jevin Yeah, boring! Who's going to click on that article? I want the predicting death article, give it to me!
Sandra So just to clarify there. The doctors were predicting at 65 to 80 percent, and the algorithm was at 85. So not really a big difference there, is it?
Jevin That's right. And in how you interpret that extra 5 percent matters a lot, too. But like Kai mentioned, because their methods aren't open, it's hard for us to question. But given the hundreds of papers that I've reviewed, and many other people have reviewed in this space, there are always problems with these kinds of really bold claims.
Sandra And you've seen a few of these bold claims, right? With people saying that they're able to predict who is going to be a criminal just by looking at their picture.
Jevin Yeah.
Sandra Or their sexual orientation.
Jevin Yeah, so these were a couple examples that were so offensive that my colleague Carl Bergstrom and I had to respond to, and others responded to these examples as well. One relates to a paper that was published on the Archive in 2016 by some computer scientists that claimed, based on their results, that they could look at your face and tell whether you're a criminal. Now, be careful, both Kai and Sandra, you don't have to go into the bathroom and look what features you have in your face.
Sandra I'm not going because I'm going to get injured.
Kai Exactly, I don't want to be one of the 30000.
Jevin If you don’t want to add to that, you could be in the dark data that you were talking about. But their claim was that you could do this. And again, this was an example, we'll ignore the black box. Most of the time, it's never where the problem is. The problem here was that they had trained on data that were mug shots of convicted criminals and pictures of those on professional websites, like LinkedIn. And I don't know about you, but when I'm standing in line to get my licence renewed, I'm not smiling. Or if I got convicted of something I can't imagine I'd be smiling and happy that I'm heading to jail. And if I'm advertising for a website, I better be smiling or who's going gonna hire me if I'm frowning? So the big difference here was that the algorithm had basically created a smile detector. And it's this kind of thing that continually happens and mistakes are made, because we ignore these selection bias issues in the training data. We don't recognise that what the algorithm is going to predict on is exactly the data we're giving it.
Kai But there's also a different problem. We get excited about algorithms that might predict a likely death in a patient. But what do you do with this then? So thinking ahead, if we had an algorithm that could predict that a given patient with 80 percent likelihood might die in the next year, what do you do with that? In an already strained healthcare system, do you use this prediction to put more resources on, or will this then be used to say 'this person, according to the algorithm, is doomed anyway, I'd better put my resources elsewhere.'?
Sandra Or the other way around? If they seem fine, do you just withhold care then, because, you know, algorithm says they're probably gonna be okay'.
Jevin The data never lies.
Sandra & Kai Bullshit.
Kai But seriously, so sometimes we're chasing the magic bullet of the predictive algorithm without actually thinking about what would we actually do with them, how would that prediction help? Because we're living our life forward, the outcome is unknown. In the study, all the outcomes were known because it was past data. So it's easy to then make those hypothetical predictions that you can judge against the real outcome. You don't know the outcome.
Jevin So to build on what you're saying, Kai, and I think it's a really important point, is that these algorithms tend to overfit for a given data set at a given time. So one of the most famous examples, you may have talked about it on the show too, of the data science success revolution was the Google Flu Trends project that everyone was so excited about, where they were saying all you need to do is look at these search queries as the data exhaust for input into predicting where the flu is going to outbreak. Well, it worked well in the first year, then not so well. The second then really not so well. The third, it was so bad. By the third and fourth year, they completely took it down. If you go to the website now, it's down. And so that was an example where we overfit. And so this like you were saying. I mean, maybe the days that they were given, they could predict, you know, 5 percent more or whatever the percentages. But what happens as we march forward in time? Do you want to be that patient sitting in the emergency room? And the algorithm says now, are not you according to our data, when in fact, you still had a 15 percent chance of living? I think I want the human to maybe help make that decision as well. It's really an unfair comparison for one doctor to be compared to an algorithm that got to look at a ton of data, too. I mean, if that doctor had enough time, I could sit down for a year to examine every one of those cases. I would say the doctor would do as good, if not better.
Kai Yeah, but isn't that the point? The algorithm can digest more and therefore it's more efficient, yada ya... Isn't that the...?
Jevin Right, it is true, like if the algorithm could actually do it, it's just when making that comparison. It's just the comparison itself is not a fair one.
Kai So the sensationalist claim to say the machine is better than the human right. That's a fallacy.
Jevin That's a fallacy. And actually, it's the same with this paper that went around saying that you could detect by looking at an image whether someone was gay or not. That also has the same sorts of problems.
Sandra The one where they took, like, Tinder shots, from the dating...
Kai From the dating website, yeah.
Jevin Yeah. This was the famous 'gaydar' paper that had all sorts of problems associated. And their claim was that you could look at an image and be able to tell whether they were gay or not. Now there could be differences in presentation that the machine's picking up, and that's possible. But being able to find special morphological features in a face that we as humans, our eyes just can't pick up, is just not true. What really is going on there is that algorithms, when given a whole bunch of data, do a lot better with conditional probability than humans. That's the big difference in those comparisons.
Kai And because the data was taken from a dating website, there's likely reasons that people who are openly gay will present themselves differently because they are complying with certain social expectations, than people who are straight. And that's probably what the algorithms then pick up on. Which does not translate to any given photograph that you would give the algorithm, that's the bullshit claim.
Jevin Exactly, and here's the other thing that's really dangerous about that. It's not that even writing the paper itself and having some researchers get, you know, upset about the problems or the methods, is that these kinds of ideas, the methods, the software that's developed then can get used by countries where being gay is punishable by death, or by countries that want to use facial recognition as a way of convicting criminals, you know, saying, 'oh, well, well there's these extra features'. And, you know, sometimes the authors will defend themselves by saying, 'well, we're just letting the world know that this technology can do this'. I don't really buy that. I don't buy it for many reasons. But I appreciate that, if it's genuine sentiment. But I think there are a lot of problems outside that kind of paper, than sort of on a whim, just putting out a paper and saying, 'oh, it's here'.
Kai And I think that's an important observation, because we've discussed a lot on this podcast in the past two years, bias and black box and all of these things, which the conversation really is honing in on now. So we understand that there are these biases and that's really good, and we're making progress. But I think we're still lacking the critical discussion around what do we actually do with these predictive technologies once we fit them into a social situation, right? Even if it could make certain predictions, how do you act upon it and who gets to act upon it? How can they be abused? How can the algorithm be used in nefarious ways, or in ways that they were never intended to be used for by the researchers that might have done the science on it?
Jevin Yeah, I think it's a really good point, Kai, and I think that is a discussion we haven't even got to yet, because we're still falling victim to the same kinds of problems, at least the constraints that machines have a lot of times. And so I think we do need to have that discussion, because there are places where machines could do a good job. Let's just say that self-driving cars really will reduce the rate of accidents and more people will be alive at year two from year one of applying, then we should be talking about it. It doesn't mean we put this under full moratorium and never do research around it. It's just that we need to have the discussions that I think you on this podcast and others force us all to have. And more than just the researchers and the tech giants need to be a part of that conversation.
Sandra So indeed, on this podcast, we've had these sort of conversations. But for instance, the conversation around the ethics of AI we've started almost three years ago, we were actually looking at all the headlines in the media and we were saying, 'well, there seems to be something missing'. There was a white space where there should have been a conversation around ethics, around bias and algorithms and whatnot. And over the last two and a half, three years, we've seen that space be populated by articles such as the one that we brought up today from The New York Times, indeed, discussing the extent to which these algorithms might discriminate against certain groups, and really having this conversation out in the open. What do you think the next one we should have is? What's the next big conversation that we're missing at this point, and how can we better arm ourselves for that one?
Jevin Well, I think wait till tomorrow, wake up in the morning and you'll find something just as discussion-worthy. But I think at least where I can say a little bit about where universities are likely going and where they seem to be moving, I think it's imperative that we train our engineers with all the technical skills that they need to go work at Microsoft or Google or any sort of tech company. But they also need to spend some time throughout that curriculum talking about the things that we're talking about right now. And I think that's happening. If you look across the world at universities, more and more of the curriculum is now asking engineers to think critically about what they're doing, to think about ethics, to think about societal implications. And I think that's going to have a big effect. I think it's important for the public, that's not necessarily of that student demographic right now, to have a good understanding of how machine learning is built. And that doesn't require an entire degree program. All you need to do is remember that these machines require data, data that's been labelled true or false or whatever that's trying to be predicted. And just that simple knowledge, I know it sounds really almost overly simplistic, but anytime you hear of a headline 'predicting death in the emergency room' or something, you can say, well, where did they get that data to train the algorithms so they could make these predictions? And then you start asking all these questions that are all the questions that every societal member, policymaker, teacher, librarian, whoever talks to the public about that, they can be the same kind of critiquer as we are in this room. So I think where it's going, at least on the education side, is integrating this into more of what engineers do on a day to day basis. Because honestly, I think universities, at least from my experience, have spent too much time on the mechanics of technology and the ability to run algorithms, to run statistical analysis and not spent enough time asking the same kind of questions and calling bullshit on what we're seeing, and not overselling like we seem to do in the Silicon Valley worlds. You know, trying to change slow moving education industries is really, really hard to do, so we need to start that now. And also, when we talk about next hot topics around this, there's a lot of talk, at least in my circles, around things like differential privacy and algorithmic ethics, if that's even a thing. These are kind of the things where it's going, but it builds off some of the same things that we're saying. But I think what we can do, the sort of deliverable or the next step that we as a community need to do and all the listeners is to question when they see these oversold headlines. How do we motivate the public to care about this? I think they should care when they're in the emergency room and they're the test case for whether they should receive care or not, based on an algorithmic decision. They should care if their investment portfolios are investing in companies that are essentially vapourware. They should care if their kids are being placed in education programs based on algorithms rather than teachers' 30 year experience. They should care when their job is taken away. I think a lot of the discussions in the political sphere right now, if you're watching the debates in the US, and there's lots of other debates going on on the changing workforce and how that is something that people do care about. And a lot of that's being done by automation. And some things are more efficient, but I think the public can't disengage with the topics around technology that for better or worse, are going to change their everyday lives.
Kai And before we end it, we have a hypothetical paper title for you which combines the two topics for today. Here it goes: "Dangerous business and toilet-training, predictive algorithms to reduce bathroom accidents".
Jevin Oh, we'll write that one together.
Kai Making the life better for the 30000.
Jevin That's right, I can already see the two minute video that will explain how we're going to save it with technology and algorithms. I can imagine a toilet sort of that knows who's going to sit on it next.
Kai And takes preventive action.
Sandra Well, happy World Toilet Day. That's all we have time for today.
Kai That's all we have time for.
Jevin Thanks for having me, this was a lot of fun.
Sandra Thanks.
Kai Thank you so much.
Sandra Thanks for listening.
Kai Thanks for listening.
Outro
This was The Future, This Week made possible by the Sydney Business Insights team and members of the Digital Disruption Research Group. And every week, right here with us, our sound editor Megan Wedge, who makes us sound good and keeps us honest. Our theme music was composed and played live on a set of garden hoses by Linsey Pollak. You can subscribe to this podcast on iTunes, Stitcher, Spotify, YouTube, SoundCloud or wherever you get your podcasts. You can follow us online on Flipboard, Twitter or sbi.sydney.edu.au. If you have any news that you want us to discuss, please send them to sbi@sydney.edu.au.
Close transcript