Uri Gal
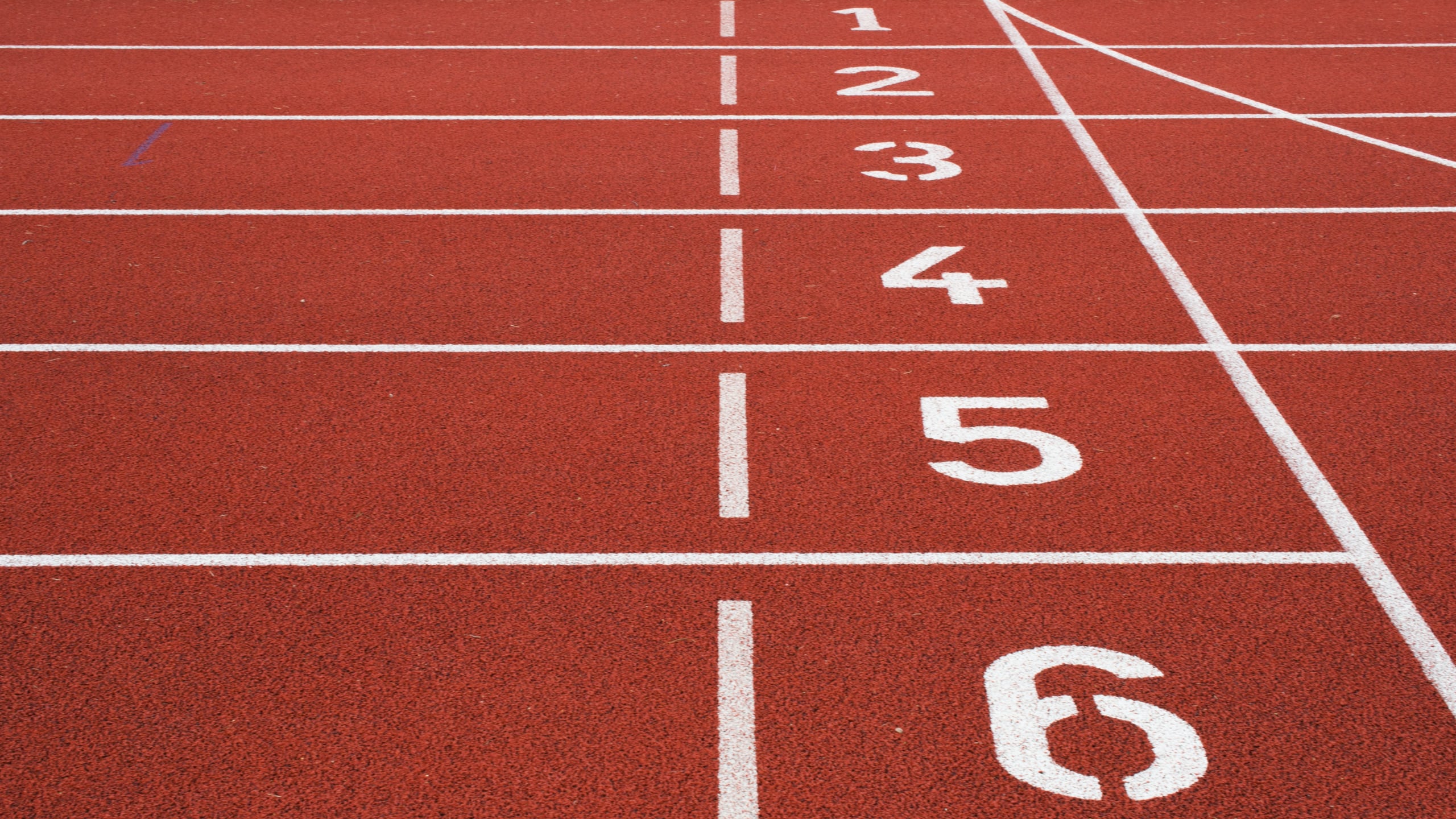
What businesses can learn from sports about using algorithms
Replacing human decision-making with algorithms seems to make sense. People tend to rely on unreliable cognitive shortcuts, get fatigued or distracted, and can be swayed by subjective opinion and inter-personal alliances.
On the other hand, algorithms are bearers of encoded logic which consistently execute pre-determined decision criteria. Therefore they are immune to emotional influences; they can rise above social relationships to objectively analyse data and optimise decisions.
But, while the application of algorithms has produced remarkable outcomes in the sporting world, this success does not fully translate to the business world. The reason is rooted in the different levels of complexity of these environments.
Algorithms in sports
One of the earliest and most successful applications of algorithmic thinking has been in professional sports. It was in the middle of the previous century when American baseball teams started using player statistics to make decisions such as which players to hire, develop, and trade.
Known as Sabermetrics, this analytical approach has since expanded to other sports and grown in sophistication.
Today, massive amounts of athlete data are collected through elaborate camera systems and wearable devices to create increasingly refined performance metrics that coaches can leverage in real time. This allows coaches to decide when to rest a player, which combinations of players are most effective, or how many points their key players need to score in order to win a game.
The profound impact of this algorithmic approach is evident in that many professional teams regard “sports analytics” to be crucial and view algorithmic innovation as key to their success.
Can the success be replicated in the world of business?
Some of the best known companies in the world utilise algorithms to enhance consumer services and optimise internal processes.
Walmart’s e-Commerce arm, Jet.com, employs algorithms that adjust prices based on the items in a customer’s checkout cart. Amazon and Netflix’s touted recommendation systems are themselves algorithms applied to vast quantities of customer data.
Algorithm-based chat bots are now commonplace in the banking and insurance industries. Uber’s pricing and ride-allocation systems are exclusively managed by algorithms that match ride demand and supply in different geographical areas in real time.
Despite their widening adoption, algorithms can have unintended negative consequences. For instance, Google, Facebook, Twitter, and Instagram have all come under fire for employing algorithms that promoted racist and abusive behaviour.
Some hiring algorithms have been shown to be racially biased whereas others denied loans to eligible applicants. In another example, rogue algorithms led to the firing of high-performing teachers.
These failures occurred not simply due to poor algorithmic design, but because of unanticipated interactions between algorithms and their complex environments.
Compared to sports, business environments are equivocal, unstructured, and dynamic. There are many possible measures for organisational success (profit, growth, market share, stock value, etc.), which cannot be easily traced back to the performance of individual employees. In fact, there are multiple dimensions along which employee performance can be assessed. Many of them are not readily observable and can be measured in various ways (engagement, commitment, innovation etc.).
Additionally, a lot of industries are characterised by hyper-competition, where disruptive innovations can render existing strategies obsolete and require rapid and profound technological and organisational changes.
Sports and business are different worlds
On the other hand, sporting competitions have a small and finite number of outcomes. The rules that govern athletes’ actions are known, accepted, explicitly enforced, and stable. Success is easily defined in terms of points, wins, and championships. Individual and team performance indicators are unambiguous and can be calculated from direct observation (points scored, hits made, assists per minute, punches per round, etc.), and their importance can be measured in terms of their relative contribution to the success of the athlete or team.
The relative stability, simplicity, and predictability of the sporting environment make it suitable for algorithmic technologies. Such technologies aim to emulate human decision-making by generating options within a well-defined problem area, identifying criteria to evaluate them, assigning weights to each option, calculating their scores, and choosing the option with the highest score.
This process faithfully mirrors decision-making processes made by team managers and coaches and can improve them due these technologies’ ability to process and analyse large datasets (a basketball coach must select five starters from his roster. The number of options is limited and his choice can be optimised by using an algorithm that analyses individual player performance data against the opposing team’s performance data).
However, research has shown that in complex, ambiguous, and dynamic conditions, such as those that characterise businesses, people engage in naturalistic decision-making. Here, people do not use algorithmic strategies to generate utility estimates for different courses of action.
In fact, no explicit action of making a decision is taken. Instead, people perceive a situation through pattern-matching against previous experience. Based on this classification, certain actions present themselves as plausible because they have been applied in previous similar situations (“I have a feeling this employee is in distress. I should call him in for a meeting”).
Because naturalistic decision-making involves little explicit deliberation and is mostly intuitive, current algorithmic technologies cannot reliably replicate it. This explains the mixed results from applying these technologies in business.
While we should not entirely exclude algorithmic technologies from business decision-making, companies would be well-advised to apply them to support analytical decision-making rather than replace naturalistic decision-making.
This article was originally published on The Conversation. Read the original article. Review the series on Algorithms at Work. Please refer to The Conversation’s republishing guidelines before republishing this article.
Image: Austris Augusts
Share
We believe in open and honest access to knowledge.
We use a Creative Commons Attribution NoDerivatives licence for our articles and podcasts, so you can republish them for free, online or in print.