Sandra Peter and Kai Riemer

Cathy O’Neil and weapons of math destruction
This week Sandra and Kai are joined by a special guest, mathematician and algorithmic activist, Dr Cathy O’Neil, who shines a light inside the black box thinking that hides the biases, flawed design and sometimes ill intent that can twist a program into a weapon of math destruction.
Algorithms are part of the invisible infrastructure that makes the world go around: Every day we are impacted by algorithmic process. What you see online, approved for a job interview or turned down for a bank loan – many of these outcomes are driven by mathematical processes far removed from human decision making.
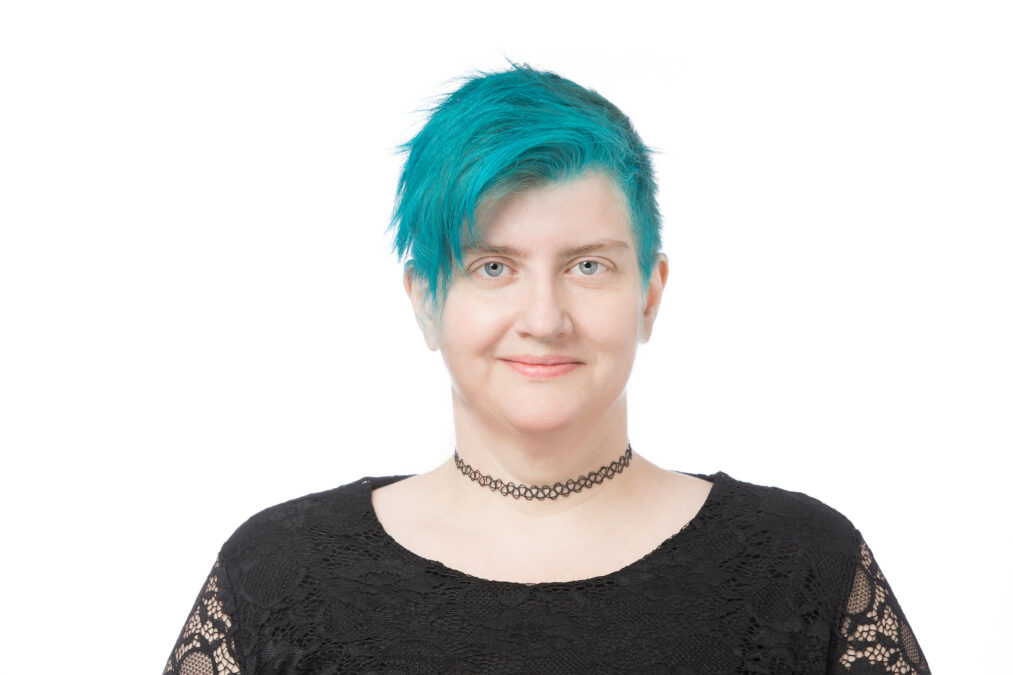
Our special guest
Cathy is a US mathematician and data scientist. She has written several books on data science including the best-selling Weapons of Math Destruction.
Cathy blogs at Mathbabe.org
Cathy O’Neil’s 2017 Ted Talk, ‘The era of blind faith in big data must end’, has been viewed more than 1.2 million times.
Other stories we bring up
Americans need a data bill of rights
A Hippocratic oath for data scientists
Are algorithms more dangerous drivers than humans?
The Ivory Tower can’t keep ignoring tech
Algorithms can improve the criminal justice system
The danger of relying on data analytics
How to teach a computer what ‘fair’ means
What’s next for humanity: Automation
Crime predicting algorithms no better than untrained humans
Don’t make AI artificially stupid in the name of transparency
Facebook patents an AI to determine social class
Algorithm with social skills teaches humans to collaborate
AI is the weapon of the next cold war
This program was produced by Jacquelyn Hole and the sound editor was Megan Wedge.
You can subscribe to this podcast on iTunes, Spotify, Soundcloud, Stitcher, Libsyn or wherever you get your podcasts. You can follow us online on Flipboard, Twitter, or sbi.sydney.edu.au.
Our theme music was composed and played by Linsey Pollak.
Send us your news ideas to sbi@sydney.edu.au.
Dr Sandra Peter is the Director of Sydney Executive Plus and Associate Professor at the University of Sydney Business School. Her research and practice focuses on engaging with the future in productive ways, and the impact of emerging technologies on business and society.
Kai Riemer is Professor of Information Technology and Organisation, and Director of Sydney Executive Plus at the University of Sydney Business School. Kai's research interest is in Disruptive Technologies, Enterprise Social Media, Virtual Work, Collaborative Technologies and the Philosophy of Technology.
Share
We believe in open and honest access to knowledge. We use a Creative Commons Attribution NoDerivatives licence for our articles and podcasts, so you can republish them for free, online or in print.
Transcript
Disclaimer: We'd like to advise that the following program may contain real news, occasional philosophy and ideas that may offend some listeners.
Intro: This is The Future, This Week on Sydney Business Insights. I'm Sandra Peter and I'm Kai Riemer and every week we get together and look at the news of the week. We discuss technology, the future of business, the weird and the wonderful and things that change the world. Okay let's start. Let's start.
Kai Riemer: Today a special edition of The Future, This Week.
Cathy O'Neil: My name's Cathy O'Neil, I'm a mathematician and data scientist.
Sandra Peter: I'm Sandra Peter, Director of Sydney Business Insights.
Kai: I'm Kai Riemer professor at the Business School and leader of the Digital Disruption Research Group. This week Sandra and I are on semester break so we have something special in store for you.
Sandra: We're bringing you a chat we've had with a special guest algorithmic activist and math babe Dr. Cathy O'Neil.
Kai: Cathy is the author of "Weapons of Math Destruction" a book that we have mentioned a number of times on the podcast before.
Sandra: In a world where algorithms are increasingly controlling the processes that run our lives, Cathy is a bit of the canary in the artificial intelligence coal mine. Algorithms are invisibly making decisions that impact virtually every aspect of our lives whether that's screening job applications or assessing how we're performing as employees and who amongst us should get a bank loan or shouldn't get a bank loan. And most people have little understanding of how these programs reach those decisions. But Cathy does. Cathy used to be a big data insider. She was writing algorithms she thought were supposed to remove human bias from important decisions and make the world a fairer and more equitable place.
Cathy: I was a total believer that math could solve these problems. By the time I left, I was really truly disillusioned and I was like this is not a math problem. This is something else.
Sandra: That something else was her realisation that too often big business and government agencies are using biased algorithms that are...
Cathy: Increasing inequality, it's sending unlucky people down a tube and it's pulling lucky people up another tube and it's doing that in a cumulative way throughout their lives to an entire society.
Sandra: So Cathy quit her corporate job and became a full time algorithmic activist.
Cathy: I feel like we're living in a naive little bubble and I want a little bit of blood on the floor so that people are like oh wait there's problems and we need to address them.
Sandra: By "we" Cathy means us, all of us. She has no confidence that left alone big business will do anything to fix the problems of silent bias.
Cathy: I don't want to be histrionic but like points to the sort of lack of education of ethics and of just pure education among the people who are running the big tech companies.
Sandra: Cathy O'Neil writes about her concerns on her mathsbabe blog.
Cathy: They should be asking the same kinds of questions like is this better than the human process it replaces? Is it going to destroy a bunch of people's lives. For whom does this algorithm fail?
Sandra: She'll help demystify what an algorithm is.
Cathy: An algorithm is, I like to say, something that we actually do in our heads all the time.
Sandra: And her ideas about how to build safeguards into the systems are upsetting some of the sectors' bigger players.
Cathy: Algorithms need to be vetted - especially the algorithms that could potentially violate people's rights or close off opportunities that they deserve or screw up democracy. Those algorithms should be vetted by an FDA for algorithms.
Cathy: Hello.
Sandra: Good morning. Good evening Cathy. How are you?
Cathy: Good.
Sandra: We really appreciate you taking time in the evening to be with us. It's the morning here so we're here with a cup of coffee. Next to me I've got Professor Kai Riemer who is my co-host on The Future, This Week. Big fan of yours as well.
Kai: Hello Cathy.
Cathy: Hi.
Sandra: I want you first to tell me a little bit about yourself. You're this self-described maths nerd with a PhD in mathematics from Harvard. Then you were all set to have a brilliant and financially rewarding career in finance or in academia but something derailed all that and for those of us who have read your book "Weapons of Math Destruction" know what changed trajectory but to some of our listeners who might be slightly intimidated by a math babe can you tell us a little bit about how you got from regular maths geek to irregular maths activist?
Cathy: Well you know it's not completely clear to me how it happened but the story I tell myself is that I still love math, I still love the kind of math I did back when I was an academic mathematician, I love number theory. I still think it's a very beautiful crystalline abstract mathematics which is to say it's almost an art rather than something that is sort of relatable and important in the real world and I think as much as I loved it I kind of wanted to feel more part of the world. But I'm a kind of impatient, provocative person and I wanted more interaction really and I wanted more than you know 10 people to read what I wrote. Not that I knew what I was doing because I didn't, I just want to make it clear that I decided to get a job outside of academics and I looked around and the job in a hedge fund was one of the only jobs that paid well that was available to me. So I spent two years at the hedge fund and then I spent two years at a different place in finance. I worked at a company that was in charge of portfolio risk management, called Risk Metrics and I actually went there with a very specific precise goal of helping them double down on math. By the time I went there it was 2009, it was the crisis's full fledged and I had the impression that the risk models hadn't been good enough and we needed to make them better and I was like oh maybe as a mathematician I can go help them make the risk models better. So I spent two years at that firm trying to improve the risk models specifically on credit default swaps which were one of the most problematic sources of risk during the crisis. You know even then I was a total believer in a certain sense I was a believer that math could solve these problems. By the time I left I was really truly disillusioned and I was like this is not a math problem. This is something else.
Sandra: And what was that something else?
Cathy: Basically a political problem. By the time I left I had enough experience with the big banks that were too big to fail, that were being propped up by the government support, they didn't really care about their risk. That was kind of my lightbulb that the risk models were window dressing and the stamp of approval that we were giving them on a daily basis was meaningless because they didn't even look at their risk. They didn't care about their risk and for that matter they didn't want to know what their real risk was and particularly my work on understanding credit default swap risk was ignored for so many reasons but one of those reasons I would argue was that the way I wanted to model the risk which I thought was more reasonable than the way we had been modelling it, it made the risk look bigger and they were just like oh that's not information we want to hear so we'll ignore that. And that was kind of the way it worked in finance. You ignore the bad news and you accept the good news and it's deep. The way I look at it at this point is that there's two different kinds of algorithms in finance. One of them is where you actually want to be accurate and the other one is when you want to be inaccurate and if you want to make profits if you're trying to anticipate the markets you want to be accurate because you want to make money and accuracy means more money but if you're trying to anticipate risk you want to be inaccurate because the more you underestimate your risk the better your profit looks compared to your risk. So you actually want your risk models to be bad and that is a deep, deep bad incentive in the whole system and I don't think that's gone away.
Sandra: You've moved from being disillusioned with this state of affairs to actually doing something about it. How did you come to write the book?
Cathy: So I've describe my journey of disillusionment within finance. I had a kind of similar but much faster journey of disillusionment once I entered data science. The people I was surrounded by were very much like Silicon Valley kool aid drinkers which is to say they were just super excited about big data and algorithms and machine learning and they thought this is the newest thing and we're going to make the world a better place and they were just super fascinated by their own tools. And I took a look at it and I was like oh wait a second this is kind of reminiscent of the way we are excited about tools in finance and that didn't end well. So I was kind of, I think we call it a Debbie Downer, I was kind of this person who was like wait a second this might go wrong. Wait a second this algorithm might be embedding racial bias. Wait a second we're optimising to the wrong definition of success or we're maybe even creating a feedback loop. The work I was specifically doing in my company was relatively benign. It was just choosing what kind of advertisement to show to people. But the more I thought about it the more I realised that even advertising in extreme cases could be predatory.
So I quit my job to sound an alarm about this kind of problem that algorithms allow us data scientists who are well educated and basically lucky people, smart people mostly white people mostly men. It allows us to create a system that works for us that seems scientific, that seems authoritative. It seems mathematical trustworthy but is actually just doing exactly what we tell it to do in giving us an advantage.
Kai: So is data science a misnomer?
Cathy: I think so. My new short term goal is to question the validity of the concept of data science itself. Is it a science in particular? I feel like no it's not because I feel a true science is something that is held up to inspection. We have hypotheses and we test those hypotheses with scientific frameworks whereas data science as it stands is just a bunch of people believing that AI is perfect and not testing it, not asking for evidence, not saying hey you've just replaced this human process that was complicated and sticky and political with a blackbox algorithm. Show me evidence that it's working. Show me evidence that it's at least as good as what it replaced. We don't even ask those questions. So if we don't ask for evidence then in what sense are we talking about science?
Sandra: Cathy, can I ask you to give us your best most easy listening definition of what is an algorithm?
Cathy: I like to say it's something that we actually do in our heads all the time, that some algorithms are formalised in computer code but they don't need to be.
So for example I think we all perform algorithms when we get dressed in the morning. We look at the available clothes (that's data), we think about the weather forecast (that's data), we think about all the outfits we wore in the past on days that had weather like this (that's more data) and what we're trying to do with all that historical data and the current state of data is we're trying to predict something and we're predicting usually a success. We're saying is this day going to be successful. If I wear this outfit will I have a successful day and of course we decide what a successful day looks like - it could be are we going to be comfortable? Are we going to stay dry? Are we going to be sufficiently warm? Are we going to look attractive? So to answer your question an algorithm is a process by which we use historical data to predict future success. And we do it by looking for patterns of success where say what in the past led to success and can I recreate a similar situation now so that I will be able to predict this similar situation will lead to success in the future. So by dint of my definition just now you'll see that algorithms are inherently backwards looking. They inherently are assuming that whatever worked in the past will work in the future which isn't to say that's true but it's just that's what they have. They have historical data and that's what they're going to go with. You know in many cases it's true but the other thing I wanted to point out about that definition is that it's extremely subjective like I'm making all sorts of choices. For example I only remember certain historical information, I haven't actually done it very systematically.
I haven't written down every single time I wore these pairs of pants but I might have specific memories of this pair of pants that were either good or bad, that was sort of like be tantamount to curating the data. So in other words it's not necessarily a particularly scientific thing. Now you might think that when we do it with algorithms with computers then it becomes much more scientific and in some sense that's true. You can be consistent in some ways but throughout my book I've found examples where we think we're more consistent than we actually are and that we actually are collecting data and using it as if it's a perfect proxy for the past but it's actually quite imperfect if not blind to certain kinds of problems. And the other thing that is subjective besides the data is our choice of success. So I might choose whether I stay warm as my definition of success for days' clothes, other people might choose whether they stay comfortable and yet other people might choose whether they feel attractive. And those are perfectly fine choices but the point is that they're different choices and if you optimise to that choice on a daily basis you will be wearing very different outfits on a daily basis. So the final point I'll make is that when you allow someone else to build an algorithm they typically do all their curating of the data, they typically choose the definition of success and they typically choose a definition of success that benefits them not you. So it is inherently a question of power. Whoever builds the algorithm chooses the definition of success and chooses the way to train their data. So for example Facebook optimises its news feed algorithm, what it's really doing is choosing a definition of success and that definition of success is you stay on Facebook longer. And when you think about what that looks like staying on Facebook longer, engaging more with more articles getting addicted to the experience of Facebook all of that of course is highly correlated with their profit, that's why they choose that. So they choose to optimise to a success which is correlated to profit. But what it also does is it privileges outrage. So you're going to more likely stay on Facebook if you get into a really long difficult conversation with someone about an outraged topic in particular it doesn't optimise to truth it doesn't optimise to short efficient conversations, it doesn't optimise to all sorts of things that we actually would prefer over engagement and outrage. So when we give Facebook the power to secretly choose a definition of success and without conferring with us we're giving them power over us and our attention. And that's just one example of literally every algorithm. I would say that almost every algorithm that is really important that's used in a scaled way across the nation the people who built that algorithm have sort of secretly decided what they're optimising to without really discussing it with all the stakeholders in question and that is the biggest problem with bad algorithms.
Kai: Cathy, is it fair to say that when you use the word algorithm you use it as a shorthand for machine learning because traditionally algorithms are quite procedural and explicit, they are auditable and easy to understand whereas what we're seeing now with machine learning they're quite different. Do you want to elaborate on that distinction?
Cathy: I will elaborate on the distinction but I don't actually think that's what I'm doing. I use algorithms very loosely to mean using historical information to predict future success. I would also argue that almost no algorithm is actually easy to understand. I think even the ones that people think are easy are not actually easy so maybe you could tell me a little bit more about what you think is easy about non machine learning algorithms. I'll grant you that machine learning algorithms themselves are very difficult to interpret and in fact that is an additional problem in the sense that the people who build machine learning algorithms don't know how they work or why they work.
Kai: Easy is probably the wrong word. The distinction I would make is between deterministic and non-deterministic as in a set number of procedures will for the same output always create a predictable output so we can have hypotheses about what the output will be. That has changed markedly with machine learning.
Cathy: Can you give me an example?
Kai: The algorithms we use in planes for example to fly the planes we can audit them and we can be fairly certain that under the same circumstances the output will always be the same. Machine learning algorithms change all the time as they learn from their inputs so that same robustness is never given, is it, which makes it a black box. It's not a black box because no one allows us to look into it but because they are internally such that we can never associate a new input with a definite output.
Cathy: I think what you're really talking about is complexity. You're focusing on an example where it's simple and you're saying it's not the case that really complicated things like Google search or Facebook newsfeeds are simple because they're changing all the time and that's of course true. But of course air traffic control systems are complicated enough because they have enough different airplanes involved that they are not simple and yet they're composed as a concert of lots of simple things. I don't think it's the nature of machine learning that's at issue here I think it's the nature of complexity itself.
Sandra: Cathy can you tell me if there are places where you think algorithms work or where it's a good idea to have them and then can you take us to the heart of your arguments about the dangers inherent in the use of algorithms.
Cathy: Sure and I didn't mean to disagree entirely with the previous question because first of all to answer your question I think algorithms are going to happen, they're the future. I don't think we can turn them off and we can stop using them. And I also am not against them. I don't hate algorithms. I just think that going back to my previous point that we haven't bothered to check whether they're working well and we haven't put the science into data science and so the other point I'll make about the previous question is machine learning algorithms in one sense are uninterpretable by their makers and they are too complex in many ways to really understand fully but that doesn't mean we can't audit them. In fact I think we absolutely must audit them. And when I say audit I don't mean totally understand, I mean things like understanding the extent to which they are legal or they are fair or they have negative externalities or they are racist or sexist.
These are questions that will be probably difficult to answer ever on an individual basis but on a statistical basis in an aggregate sense I think we can approach them even though they're uninterpretable lockbox algorithms. So I guess the answer to the question is I think algorithms can be much better than they are now and that we must make them better. We must hold them accountable because they are going to be the future. And moreover every industry at least in the United States already that has repetitive bureaucratic decisions to make are replacing those bureaucratic decision making processes with algorithms. So we are literally going to have algorithms in every industry and the question that we're going to have to ask ourselves is are we going to let them run free without checking to make sure they're working or are we going to actually check to make sure they're working and I think we have to check because otherwise the entire fabric of our society will be a free for all. The things that I worry about of course are that number one: these things aren't fair, that they're destructive, that they are actually having systemic negative effects on the people that are being targeted because most of the algorithms I worry about are essentially scoring algorithms, they score individuals to decide whether those individuals are worthy of opportunities. Are they worthy of a job? Are they worthy of a loan? Or of a good insurance policy? Or should they pay more for insurance or should they get higher APRA in their loan or should they get a worse job or no job or should they not get a bonus so they should get a bonus or should they get into a good college or a bad college or no college at all. Those are all questions that are now being answered by algorithms and they're not being audited, those algorithms. So they might and they probably are from my experience basically just profiling people, separating out the lucky from the unlucky and essentially making lucky people luckier or unlucky people unluckier. That is essentially what data science algorithms do. And if you think about how algorithms are in every juncture of people's lives that involves a bureaucratic decision point what that's really doing as a whole is it's increasing inequality. It's sending unlucky people down a tube and it's pulling lucky people up another tube and it's doing that in a cumulative way throughout their lives to an entire society. So the net result is we had this almost invisible force that increases inequality and it's all being done almost carelessly because we simply don't double check that algorithms are working well.
Sandra: You have quite a few very very good examples in your book. Can you walk us through one that actually illustrates how we inflict these injustices to the most vulnerable people and how these actually go unchallenged?
Cathy: I have a chapter in the book about predatory online advertising for for- profit colleges, also a very similar system for payday loans and there's incredibly well-developed data warehousing and targeting systems on Google and Facebook, this is how they make their money, that will help advertisers target poor people who are also naive enough to fall for the come-ons from a for-profit college or they're poor enough and desperate enough to ask for a loan or similarly they're addicted to gambling so they're going to fall for a come-on from a gambling house etc. That's a whole industry which is the lifeblood of online advertising. I have examples of a teacher value-added model. This is a way of assessing teachers, that is secret, that in some places in the country results in losing your job. And yet it's almost a statistically random process. In other words a given individual might get a, and I've found people who have gotten a 6 out of 100 one year and then the following year they'll get a 96 out of 100 so they'll think are the worst teacher in the world and then they'll go to thinking they're the best teacher in the world and they haven't changed the way they teach. That's not an anomalous example because there have been aggregate statistics done and it's found that the correlation for scorers for the same teacher for the same subject even in the same year are very low. It's almost a random number generator and yet it's being used for these high stakes decisions. That's just a bad bad algorithm but the reason it increases inequality is because that algorithm is not used universally it's used essentially in poor minority neighborhood school systems which means they are treating the teachers in those poor minority school system districts much worse than they're treating teachers in richer more affluent whiter suburbs - that overall has an effect which is bad for poor kids. That's one example.
Kai: Cathy, more and more examples that we learn through your work and other people's work about the inappropriate use of algorithms and the use of commercially in confidence algorithms where they are black boxes not necessarily because we couldn't understand them but because no one will actually give us access to the algorithms. I want to ask a bigger question - why do we use algorithms in those situations? Why do people reach for technology when clearly it often isn't appropriate to do so? What's your view?
Cathy: That's a great question and I think the answer is multifaceted and it's contextual so I think the first answer is that some people do it simply because it does save them money or it makes their process more efficient, it allows them to hire fewer people in human resources, replace an entire group of human resources people with an algorithm. It's just cost saving. Now of course that's accepting the good news by ignoring the bad news. The very short version is that it's not just a marketing trick that other people think that algorithms are perfect. I think there's a lack of education that the people who are buying these algorithms themselves are tricked by the marketing of big data as perfect. That's one thing. The second thing is we simply just don't think through longer term consequences so Facebook probably didn't expect the negative externalities of its algorithm. They didn't know in advance or think in advance that they would be privileging outrage and privileging conspiracy theories. They should have been thinking about that but they weren't because they were Silicon Valley companies and Silicon Valley companies think short term. Then there's another sort of category of why people adopt algorithms and I will speak closer to the teacher algorithm that I talked about before the teacher value-added model. I think that, and I don't want to sound cynical because I think this is a real reason, even though it sounds like a cynical reason.
I think that was an example where people were just sick of having a sort of never ending complicated conversation about what makes a teacher a good teacher because people disagree. It's a really hard question to answer and so instead of having that conversation yet again they're like well let's use an algorithm that will tell us the answer and what's good about that is we don't have to have this silly conversation anymore. And also as a by and by nobody in particular will be responsible for it if the algorithm is wrong. So there's that secondary thing which is there is a lack of accountability which is actually in many cases in a bureaucratic system a bonus for the people who otherwise might be held accountable. In other words if they stuck their neck out and said here's my definition of a good teacher I'm going to stick with it and this is the rule from now on and everyone has to follow my rule, if there ends up being problems with that rule then they're going to be the ones who get fired or at least get blamed whereas if you have an algorithm it's a black box and if there's a problem they can point at that black box and say hey it wasn't me it was the black box. So I'm not sure that was an explicit goal of people when they adopted algorithms, but I do think that seems to be a very convenient feature of the algorithms and in part why algorithms are not removed even when they have problems.
Kai: You've given us one of the three arguments you make in your book about what is wrong with those algorithms which is the outcome that they lead to injustices. You mention two other ones which is that they are black boxes and they also lack feedback loops. Do you want to elaborate on this for our listeners?
Cathy: Right. So there's two different feedback loops going on. Let me carve out the difference between the two. One of them the feedback loop I talked about already was the increasing inequality, so how it makes unlucky people unluckier or lucky people luckier, I think of that as a destructive feedback loop which I think is going on and is a problem. But there is another feedback loop which is a good positive echo system for an algorithm itself and like a successful algorithm needs a feedback loop which is to say a positive data feedback loop. And my favorite example of a system that I think is constructed very very well for the algorithm for the purpose it's serving is the Amazon checkout line. Now I'm not trying to make the case that Amazon is the best thing that's ever happened to the world because I actually am very afraid of Amazon and I think in 10 years it might be the only company and I think that that's something we should be worried about. But I will say that the use of data is extraordinary and it's admirable and in particular what I mean by that is they follow the data, they follow the successes, they follow the failures, they learn on a daily if not second by second basis.
So if they show me a product that they think I might want to buy and I do buy it then they can show me other products like that. That people who bought that also about this or based on your history you're going to buy this and they learn they learn from what I do what I don't do and from what other people do and what other people don't do. If I ignore a product that they've offered me they learn from that too - they're like oh you didn't care about this oh we're going to learn from our failures and learning from your failures as well as your successes is something that most of the algorithms I talk about in my book fail to do. They actually fail to have a feedback loop like they're learning from mistakes and successes. There's almost always a fail to feedback loop in that sense for these terrible algorithms and for example for the teacher algorithm there's no feedback loop there at all. There was no way for teachers to be measured with this value-added model which again I said it's almost a random number generator and there's no way to test that against some other trusted ground truth - is this teacher good or not. They simply didn't have a secondary way of measuring the teachers. So it became its own reality and that's no good because that means that you can't tell if something has failed. You can't tell if something has succeeded and you cannot learn from your failures and successes. So you've filtered people out and they're gone and you don't track them and you don't learn from them. You don't learn from the mistakes that you have made. So that's called a false negative. You've never learned from the false negatives.
Now having said that you do learn from false positives. False positive in the context of hiring means that you hire somebody you think they're going to work out but they don't end up working out. So you do learn from false positives and what ends up happening when you're learning from false positives in the context of hiring is you restrict more. You have a even stronger filter because you're like oh we've now learned that people like this don't work out. So you turn up your filter to have even fewer people show up.
Kai: Do you want to elaborate briefly on what we mean by a black box?
Cathy: Sorry I had to take a drink.
Sandra: We're joining you with coffee.
Cathy: Oh ok great. What I mean by a black box algorithm is an algorithm that we don't understand, we don't necessarily even care about sort of the thought process within the box, within the algorithm. We don't know what the source code is we don't understand it, quote unquote. We just know we can track what happens to inputs like we could see what happens what the output is. I mean black box makes it sound pretty unintelligible and in some sense it is like we're not going to understand it. I just want to say that you can learn a lot about a black box algorithm if you have access to it and you have access to inputs and you could explicitly feed certain types of inputs and change them a little bit see how the outputs change. There are ways to sort of decipher certain aspects of an algorithm even a black box algorithm if you have enough access to it.
So it's not completely monolithic and inscrutable even if it's called a black box algorithm.
Kai: Is that a technical problem or is that a political problem because I can envision systems which are making decisions about people that are based on a decision tree which if we had access we would be able to decipher but quite often in public service or in commercial situations that access is never allowed vis a vis systems that are just too complex for even the developers or owners to understand.
Cathy: Yeah I mean it's a great question and there are laws - there's a law in the United States that if you apply for a credit card and you're denied a line of credit then you deserve an explanation, that you can demand an explanation and that they have to give you one. And what that ends up meaning is that they have to use a decision tree essentially they have to come up with a way of explaining to you why you failed to get your credit card. So in that example in that context they've decided that explainability is an important enough goal that they can make it a law and that restricts the type of algorithm that is used by the practitioners. Now having said that I would argue, and you didn't ask this question but, there is a new law coming into effect in Europe called GDPR. Part of that new law seems to imply that Europeans who are subject to these kinds of scoring systems might have the right to demand an explanation for a lot of different kinds of scores not just for credit by the way the credit law thing is in the United States not in Europe but my larger point is that explanations per say aren't necessarily useful.
So on the one hand it's kind of cool that you could sometimes get an explanation because you know wow it's a black box and yet I got an explanation. On the other hand I come from the world of hedge funds and in the world of hedge funds, if you work there for a while, what you've learned how to do very quickly is to follow the letter of the law without the spirit of the law at all. So when I hear something like oh you have to provide an explanation I immediately think to myself oh I can provide a meaningless explanation. Define explanation. And if you think that sounds cynical which it does because I am kind of cynical about this particular thing, just recently Bloomberg had a piece about the feature that Facebook added recently that allows users to click on the question "why am I seeing this ad?" basically an explanation from Facebook about why you're seeing a particular ad and the explanations were meaningless. They were like oh because you're at least 13 years old and you're in Italy. The particular example of that, by the way, the user had this very I hope I'm saying this right but it's along the following lines they have a sort of like progressive left politically, but in order to test their hypothesis they liked a couple very strong right wing political groups on Facebook and then presto it changes a couple hours later on Facebook and they were shown a right wing ad. So they had a particular idea in mind like I know why I'm seeing this right wing ad but when they clicked on it they're like oh you're 13, you're live in Italy. And I was like no that is not why. I'm just trying to explain that it's not enough to say I want an explanation. You have to say what you mean by that. So in other words you're going to say something along the lines of "I want a meaningful explanation" or you're going to say "I want to know whether my rights are being violated". That's really what you want to know. I want to know whether I'm being treated fairly.
Kai: No, I agree.
Cathy: That's not something that I will expect anytime soon with an algorithm and that's a lot harder and that's not to say we shouldn't be demanding that we should be demanding that but I don't think it's going to be an AI demand, I think it's going to be literally a law.
Sandra: So what can be done to fix this? You've started talking a little bit about regulation in this space. You clearly don't think market is going to solve the problem. So is government regulation the only thing we're left with? You've also recently called for a data scientist to sign a Hippocratic Oath - what would that involve? And how would we make sure that that is actually a tool that works?
Cathy: So to be clear I do want a Hippocratic Oath but I don't think it's going to be sufficient because I know a lot of really good people who work at Facebook and they can't change the business model there nor can they at Google nor can they at Palantir. There's plenty of companies that just don't give power to their data scientists. So an ethical data scientist is only going to have so much effect. So I do think we absolutely need regulation. My idea is what I call a data bill of rights and it has two parts.
And I'm going to explain it with an American centric view of it because I'm trying to sell it in America but to tell you the truth this will not work unless it's an international treaty. And I know that sounds grandiose but I expect this will have to happen in the next 20 years and I am thinking along those lines 10/20 years before we can expect something like this. But I think this stuff is happening so quickly and we're going to be seeing so much damage if we don't do something in a concerted fashion that we might as well start thinking in those terms. The two different parts to my data bill of rights are essentially individual rights, on the one hand and aggregate issues on the other, and so I'll talk about the aggregate stuff first. I want to model it along the lines of the FDA in the US we have this Federal Drug Association - it's looking to see before new drugs are distributed to millions of people whether people will die when they take those drugs. First of all and second of all whether they'll get better faster than with the current medications. What I'm suggesting is that algorithms need to be vetted too, especially the algorithms that could potentially violate people's rights or close off opportunities that they deserve or screw up democracy. Those algorithms should be vetted by an FDA for algorithms and they should be asking the same kinds of questions like is this better than the human process it replaces? Is it going to destroy a bunch of people's lives? For whom does this algorithm fail? What are the longer term consequences here and how do we measure those longer term consequences?
So that's the aggregate view. On the individual rights view, what I'm basically asking for is also modeled after something which are the laws that we do have around credit scoring. So there's something called the FICO credit score in the States, widely used, it's called the Credit Report. We have the right to look at the data on a yearly basis or maybe by yearly basis we have a right to look at the data that goes into our score. And if we see the data that's wrong we're allowed to complain, we're allowed to appeal it. So I'm asking for that right to be extended to all algorithms, all scoring systems that have anything to do with our finances, that has anything to do with our livelihoods like our job, keeping our job, getting a job, or that has anything to do with our liberty because we haven't even talked about this but I devote quite a bit of my book to questions of policing algorithms and sentencing and parole and bail hearings that judges are using algorithms to help sentence people. And those are algorithms like all the other ones in my book, they're very flawed. I think they're deeply racist and they're not vetted. We do not know how they work.
Kai: Should there be areas where we shouldn't use algorithms, where we must rely on human judgement. And if so how would we decide where to use algorithms and where not?
Cathy: Well we certainly shouldn't use algorithms when they don't work. And I would argue that the teacher algorithm which I referred to as a random number generator, it doesn't work. We shouldn't be using it period.
Kai: Yeah but sometimes that distinction is not easily made right. Because in the justice systems you could argue the algorithm works because it has cleared out the backlog of cases and we're much more productive, we're making decisions faster so on some kind of metrics it works and yeah it depends on the definition of success and what working means. And some of the negative effects bias and discrimination might be backgrounded or come to be seen much much later and only through some dedicated activism. So how do we make that distinction?
Cathy: It's a great question and to answer that question I have been working with Hanna Gunn who is an ethicist, a moral philosopher and she's introduced me when I'm introducing to the world of algorithmic accountability this concept of an ethical matrix and it's exactly what you've just said, it's a very simple idea. It's just a construction, it's a matrix it has rows and columns - the rows are the stakeholders of an algorithm in a given context and the columns are the concerns of those stakeholders in that given context. So for example if you're talking about a sentencing algorithm that a judge uses a score for a criminal defendant to decide how long to sentence them based on how likely they are to return to prison, the algorithm isn't "successful" quote unquote simply because it is efficient, right. That is a concern but it's not the only concern because that is only the concern of the court system itself. That's not the only stakeholder though, the stakeholders are the court system, the judge, the defendant, the public.
And if you wanted you could separate defendants who were scored correctly and defendants who are scored incorrectly because they have different concerns and then the concerns are things like efficiency, accuracy, false positives, false negatives, justice, fairness. So if you think about this I've already given you a pretty big matrix you have to consider every single stakeholder along every single concern and that's a lot of considerations, it's a two dimensional field of considerations. The way I simplify that is I colour code the cells of that matrix and I make them green if there's not much to worry about, I make them yellow if I'm worried, I make them red if I'm very worried. And so the very short answer to your question of how do we know whether it's okay to use an algorithm at a given context is write down the ethical matrix and see how many red cells you have and if you have a red cell you probably shouldn't be using that algorithm.
Kai: Okay. Fair point.
Cathy: By the way, that's not to say how to fix the algorithm to get rid of red cells.
Kai: Oh no no no no, I agree I agree.
Cathy: I'm not saying you cannot fix it and I'm not saying you can fix it. In some sense, what I'm saying is it's complicated. We can't pretend it's not complicated.
Kai: No I agree but I'm a firm believer that there are certain things that algorithms just can't do and we shouldn't pretend that they can. So I'm probably a little bit more black and white here in the sense that I fundamentally think human judgement is of a non algorithmic kind but it is very helpful to have a mechanism to actually foreground that and to show people that we have a problem here. We cannot ignore this and if we don't find a solution in the meantime we mustn't use the algorithm.
Cathy: So what's an example of a context in which you think algorithms should simply never be used?
Kai: Well where we need judgement because I don't think that judgement is the kind of thing that we can formalise as an algorithm. Are you familiar with the work of philosopher Hubert Dreyfus?
Cathy: No.
Kai: Oh I think you might be interested. He's written a book "What computers can't do" and then later "What computers still can't do". He actually has a little bit of a similar history to yours because he was a concerned renegade philosopher at M.I.T. when the first wave of AI came about and this led him to be a critic of basically algorithmic formalisation of human expertise in decision making. So I'm basing some of those insights on his work.
Cathy: I am not against this idea that there are situations where algorithms will never be appropriate but every time I think I come up with an example I'm just like well you know even with the teachers I think we are very very far away from understanding formally in an algorithmic way understanding when a teacher is a good teacher. The way we're doing it now with just basing it on test scores alone is just ridiculous and we all know that. We all know teachers that were great not because their kids did well on tests alone anyway. And I can't imagine an algorithm that would be able to do that. But on the other hand I could sort of imagine specific things that could be measured.
We could certainly measure whether it a teacher engages lots of kids, we could use sensors in a classroom to do so. We could see whether kids are talking inappropriately or people were yelling and I'm not saying that's a good idea to have sensors all over the classroom. I guess what I'm saying is like, as a thought experiment since I'm a mathematician I really like thought experiments, I can't prove to myself that there will never be an algorithm that can do this.
Sandra: I want to move a little bit to talk about the future and the future of algorithms and I've got two areas that I want to take you to and one of them is China and to talk a little bit about what you see in the race between China and the US especially in terms of algorithms, machine learning, artificial intelligence, this is shaping up to be a two horse race and how do you see this in terms of long term development?
Cathy: So I have conflicted feelings about China vs. the US. On the one hand I think it's fair to say that the US is way more advanced than China is at having dossiers and profiles and enormous amounts of data collection on everybody, every consumer in the US, adult anyway, than China. So in some sense I feel like the people's sort of hysterical explanation of what China's doing with the social credit scoring systems that they're building are kind of overblown because like we have way more data on people and we also essentially use the FICO credit score as a way of measuring somebody's moral health care.
So we're doing a lot of the same things probably better in the sense of probably with more data probably at a more granular level than China. On the other hand I would say that China's stated goals for their social credit scoring systems are on their face authoritarian and deeply unattractive. And they're doing all sorts of other kinds of data collection that is deeply disturbing like I heard they were going to schools in ethnic minority areas and asking every child in the school to offer a DNA sample to be put into a DNA database just in case any of them ever commit a crime. And if that's true that's terrible.
Sandra: Since 2016 I believe every person applying for a passport needs to submit a DNA sample.
Cathy: I didn't know that.
Sandra: So now they have the largest database of sequenced DNA.
Cathy: Yeah.
Sandra: I think it's by an order of magnitude compared to...
Cathy: And they're not hiding their intentions either. They're like we're going to get bad guys and we are going to control our population and they know it and they know they're being watched and there's sort of like an explicit threat that if you say something bad about the government that not only will your score go down but your friends' and family's scores will go down. So there's like deep deep disturbing pressure that they're putting on but on the other hand when I go there I just have to come back to the United States and acknowledge that we do that too and there's plenty of people in Silicon Valley that seem to think that's a good idea. I was reviewing a book that was written by the former chief data scientist at Amazon. I reviewed it for I think Scientific American where he suggested that we measure people's social utility before deciding who should get organ transplants.
That's insane and it's completely unethical and I don't want to be histrionic but points to this lack of ethics and of just pure education among the people who are running the big tech companies.
Sandra: So if you look towards the future what really scares you?
Cathy: Well I'm conflicted about answering this question because sometimes I want to be scared because sometimes what I want is bad news to come out so that we will all be a little bit more realistic about what's actually happening. Do you see what I mean? I feel like we're living in a naïve little bubble and I want a little bit of blood on the floor so that people are like oh wait there's problems and we need to address them. Which isn't to say I want people to suffer. But I just feel like what's happening now is sort of invisible suffering that we're not seeing enough.
Sandra: What do you think a wake-up call like that would look like?
Cathy: Well I think we've had a mini wake-up call with the Cambridge Analytica stuff. I think people were kind of distracted by thinking that it's a privacy problem but not really being precise about what the harms are of lost privacy. And I think the harms of lost privacy are actually hard to disentangle and to point to and that's one of the reasons that it's going to be hard to get blood on the floor. The way I look at it is when a whole ton of people lose a bunch of personal information it's not like they all get hit at the same time and you can sort of have collective action to complain about it.
It's much more like some of those people eventually get turned down for jobs because of the stuff that's in their file but they probably are never told why they don't get that job. So I guess to answer your question what do I worry about seeing is what I worry about seeing is nothing. I worry about this not coming to light.
Sandra: Clearly the Facebook and Cambridge Analytica scandal wasn't enough to wake people up. What do you think they would need to see on the front page of the newspaper for that to be a wake up call?
Cathy: So what does blood on the floor look like? It would look like somebody figures out this algorithm is racist and it has sent a bunch of people to prison that shouldn't have been there or something along those lines or a thousand people were denied a job because they happened to be living in the wrong zip code or these people had to pay extra for their mortgage because they had the wrong URL. And by the way that kind of headline actually does happen already and not enough changes. So I don't want to be unrealistic about what is the trigger where we're all going to fix this problem.
Sandra: So you don't actually think there's something that could actually change people's minds or get people to see the magnitude of this?
Cathy: I do. No, I mean I don't want to end on a hopeless note like that. What I mean to say is it's going to be a cumulative long term effort. I liken it to how long it took cars to be safe. We didn't have seatbelts. We didn't have airbags. We didn't have crash test dummy tests for decades. People were dying. We eventually figured out we don't have to have this much destruction. We can do better than this. And we're going to have to eventually figure that out with algorithms too. I don't know the year it's going to happen but I do think it will happen.
Sandra: And I think that's a great point to leave it on. Cathy thank you so so much for being so fantastic and accommodating tonight. It's been an absolute pleasure having you on and having these conversations and touching on so many things.
Kai: Thanks Cathy.
Cathy: My pleasure thanks for having me guys.
Kai: Thanks so much.
Outro: This was The Future, This Week made awesome by the Sydney Business Insights team and members of the Digital Disruption Research Group. And every week right here with us our sound editor Megan Wedge who makes us sound good and keeps us honest. Our theme music was composed and played live from a set of garden hoses. You can subscribe to this podcast on iTunes, Stitcher, Spotify, SoundCloud or wherever you get your podcasts. You can follow us online on Flipboard, Twitter or sbi.sydney.edu.au. If you have any news you want to send us please send them to sbi@sydney.edu.au.
Close transcript