Sandra Peter and Kai Riemer
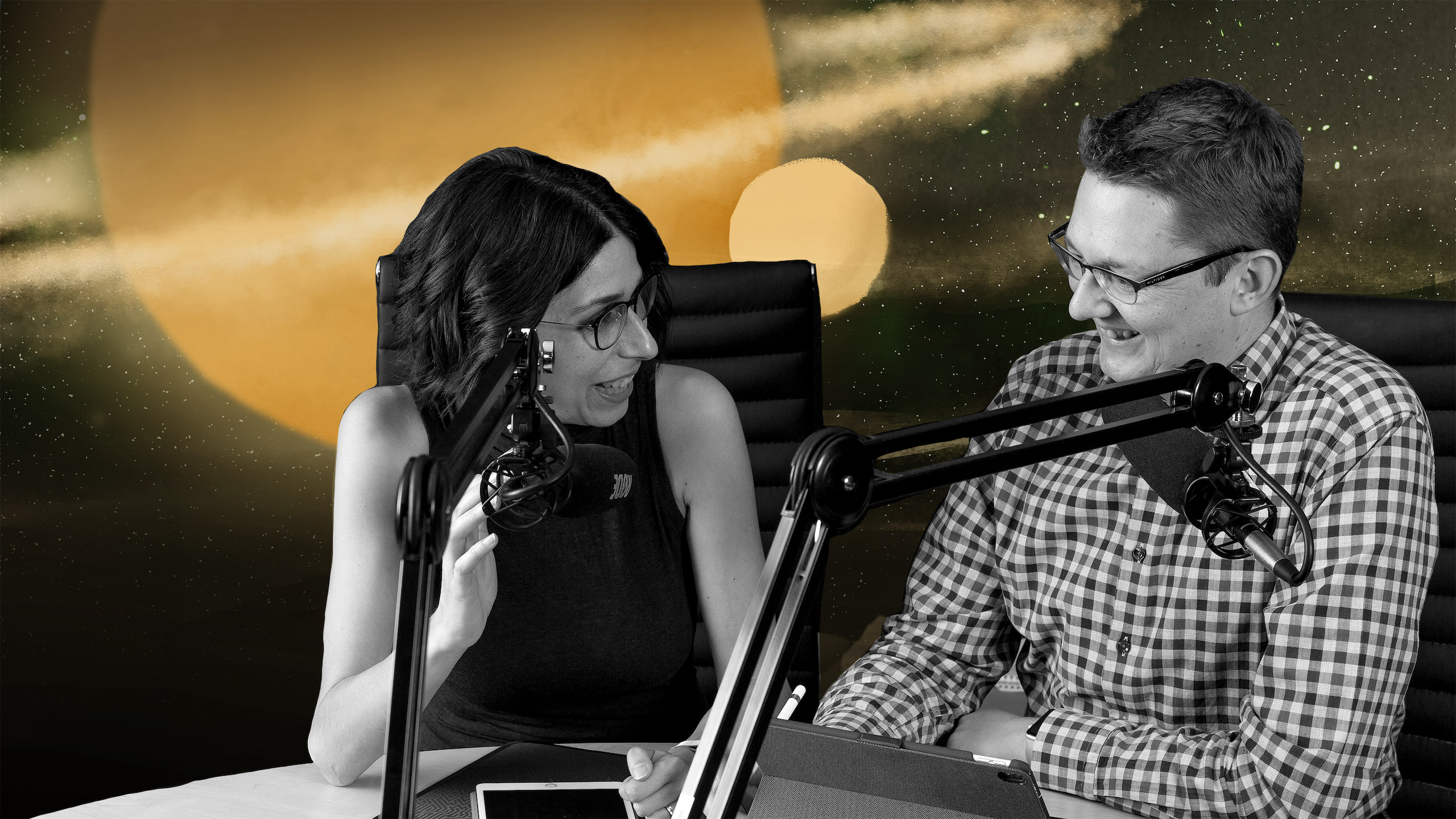
The Future, This Week 25 August 2017
This week: what’s up with the hype, computer says no, and the power of playlists. Sandra Peter (Sydney Business Insights) and Kai Riemer (Digital Disruption Research Group) meet once a week to put their own spin on news that is impacting the future of business in The Future, This Week.
The stories this week
The hype around the Gartner hype cycle
How Spotify playlists create hits
Other stories we bring up
8 lessons from 20 years of hype cycles
Why Gartner dropped big data off the hype curve
When government rules by software
Algorithmic transparency for the Smart City paper
Irish engineer who failed Australian visa English fluency test
The numbers game behind Spotify cover songs
Are Spotify’s ‘fake artists’ any good?
Spotify denies filling popular playlists with ‘fake artists’
Our robot of the week
Massive robot dance – Guinness World Records
Follow the show on Apple Podcasts, Spotify, Overcast, Google Podcasts, Pocket Casts or wherever you get your podcasts. You can follow Sydney Business Insights on Flipboard, LinkedIn, Twitter and WeChat to keep updated with our latest insights.
Send us your news ideas to sbi@sydney.edu.au
For more episodes of The Future, This Week see our playlists
Dr Sandra Peter is the Director of Sydney Executive Plus and Associate Professor at the University of Sydney Business School. Her research and practice focuses on engaging with the future in productive ways, and the impact of emerging technologies on business and society.
Kai Riemer is Professor of Information Technology and Organisation, and Director of Sydney Executive Plus at the University of Sydney Business School. Kai's research interest is in Disruptive Technologies, Enterprise Social Media, Virtual Work, Collaborative Technologies and the Philosophy of Technology.
Share
We believe in open and honest access to knowledge.
We use a Creative Commons Attribution NoDerivatives licence for our articles and podcasts, so you can republish them for free, online or in print.
Transcript
Introduction: This is The Future, This Week on Sydney Business Insights. I'm Sandra Peter and I'm Kai Riemer. Every week we get together and look at the news of the week. We discuss technology, the future of business, the weird and the wonderful things that change the world. OK let's roll.
Kai: Today on The Future, This Week: what's up with the hype, computer says no, and the power of playlists.
Sandra: I'm Sandra Peter. I'm the director of Sydney Business Insights.
Kai: I'm Kai Riemer, professor at the Business School and leader of the Digital Disruption Research Group. So Sandra what happened in the future this week?
Sandra: Gartner's hype cycle for emerging technologies, 2017 adds 5G and deep learning for the first time. So this article reports on the new technologies and the new trends that have made it onto the hype cycle and talks in particular about five mega trends that they see, artificial intelligence everywhere transparently immersive experiences and digital platforms as the big things that will shape the years to come and also tries to place, out of over two thousand technologies that the advisory firm tracks, try to place the ones that are either on the peak of inflated expectations or have actually made it to a slope of enlightenment.
Kai: So this hype cycle thing is a big thing right. It comes out every year and Gartner publishes the one on the emerging technologies which is sort of the overall hype cycle model and then they have about 80 or 90 industry specific hype cycles and it's really important for people within those industries that whatever they are interested in is listed in those hype cycles because people actually pay a lot of attention to the hype cycle so it can make and break industries.
Sandra: Since this is a big thing that's being reported on in many many companies pay attention to this, we thought today we should really talk a little bit about where does this hype cycle come from. What's on it, how do things make it on it. So what? Why does it matter and what do you do with it? And are there any challenges or problems with it?
Kai: So first of all the hype cycle is a curve. It's not really a cycle. There is no circle cycle there. So it's this curve that looks a bit like a sinus curve it's got a very steep ascent. It's got like a mountain top and then it goes downhill into a little trough and then it sort of extends into a longish plateau. That's what it looks like. And Gardner distinguishes five different phases. They start off with the innovation trigger. This is where new technologies speculative things make their way up in to our collective consciousness. Then we have the peak of inflated expectations. These are the things that are big in the media that everyone talks about.
Sandra: This is the buzz everything that we talk about, everything that we hear about in the media...
Kai:...that's where the hype happens and then it sort of falls down into what they call the trough of disillusionment. This is where things didn't quite work out in the short term, that was inflated expectations and people lose interest in those technologies.
Sandra:...and then they fail to deliver any financial benefits for what they were good for. There was a much slower adoption rate than it was initially predicted or hype.
Kai: And then slowly slowly things work their way up into the slope of enlightenment where it becomes clear what things are really useful for usually it's a much smaller application space. It's not the silver bullet but it becomes something in the end and then.
Sandra:...it starts to work for early adopters of technology...
Kai:...and it exits the curve via the plateau of productivity where things are actually being applied. So let's talk about how Gartner actually comes to place technologies along this curve. What do they do?
Sandra: Gardner looks at over 2000 technologies every year and picks a few that it places across this curve. And the big question is how does it place them along the curve and on the two axis. First let's make it quite clear that the hype cycle is a qualitative tool. There is no single measure for what they do on the Y variable which are these expectations. They use a variety of surveys and most of it are forecasts made by the analysts who work for Gartner. They look at the media, they talk to people, they listen to what people are saying and they place the technologies on the curve. So on the one axis we have expectations, on the other axis we've got time and with time we've got technologies that are about to mature that have one or two years to mature. Or they might have five to 10 years to mature.
Kai: So they actually have these little symbols that go with every technology on that curve and they suggest that those technologies move at different speeds along that curve.
Sandra: Gardner says that the strength of this is that there are no quantitative measures for anything that's going on there. There is highly experienced experts involved in making the judgement. So this is not an academic endeavour, it's a consulting company that comes up with this and what we want to point out is that while for the first part of the hype cycle, the hype part of it, there are actually indicators in the media that you can look at to see whether these technologies are indeed hyped or not. The second part is more a call on engineering or business maturity of those technologies where actually there could be some numbers that you could put behind whether or not these technologies are working.
Kai: So the question is, is this thing useful? Right. And many people would say yes it is useful because it gives an indication of what are the things to come, what are the buzz words, what are the concepts the technologies to watch out for. So that is largely about the things that are not yet on our radar. What is less clear to me is what happens with that other part of the curve where things are actually moving through the more difficult phase and then into the slope of enlightenment and the plateau of productivity and indeed, and we will discuss this, there's a bit of a problem there because the curve for this year in particular doesn't show much on that side of the curve so there's a lot of technologies placed on the upcoming innovation trigger, the things that are about to be hyped, according to Gartner but there's not a whole lot of things that are actually maturing to productivity. So why is that?
Sandra: So this is a question really about how things enter the hype cycle and how things might make their exit off the hype cycle and how they move on the hype cycle from year to year. Interesting analysis by a guy called Michael Mullany and this was published on LinkedIn last year. Looked at the fact that very few technologies actually travel through the entire hype cycle in the way that we would expect them to. And that in practice most of the really big technologies that we've seen over the last 20 years have not really moved through the motions on the hype cycle. One example was intelligent agents. This was Clippy the little paper clip in your office in your Word document that was trying to help you. That was the early mid 1990s version of intelligent assistance that we are seeing come back now. And the core technology around that the contextual reasoning is still a thing. And it's been 20 years of it going on and off the hype cycle.
Kai: Clippy. This is where our listeners get the shivers right because everyone hated it so much and countless computer monitors got destroyed in its wake.
Sandra: And it basically killed off intelligence agents for about 15 years because nobody wanted to touch it.
Kai: Nobody wanted Clippy to come back.
Sandra: Maybe that's why they don't have a face these days.
Kai: Clippy is back though. We call it Siri or Google Home now.
Sandra: Yes but they don't have a face. They have a voice because Clippy is just so burnt into our memory. But seriously, just a handful of technology things like cloud computing or 3D printing or electronic ink have ever been identified early enough and have travelled through the hype cycle which tells you we're really horrible at making predictions.
Kai: If only about five out of 200 technologies have travelled all the way across the hype cycle, it's not really a hype cycle is it?
Sandra: Well it clearly shows that quite a number of technologies, and this is something also pointed out in the article, that about 50 of the individual technologies appear for just one year and then they disappear. So many of these things are really just hype. And also we should know that quite a few major technologies have flown completely under the hype cycle radar. Things that might look really trivial in the beginning or might not be picked up in the media have been really foundational for the way we do business now and a couple of examples that are brought up there one of them is Hadoop which really was the foundation for this generation of large scale data analysis and open source. Open source as a licencing model that really led to the rise of communities for code sharing, enabled cloud computing models, infrastructure software these have been major things that have not been on the hype cycle.
Kai: And sometimes things just disappear right. So take big data - big data made its appearance in 2011 as "big data" in quote marks and extreme information processing and management and then moved up the hype cycle in 2012, 2013, it peaked 2014. It slowly moved towards the trough of disillusionment and then it was gone. In 2015 big data just disappeared from the hype cycle. Now according to Gartner there's always a reason why something has dropped and they tend to explain these things in a footnote somewhere in their report. And we found an article that actually outlines why they dropped big data in 2015 but it doesn't really satisfy - what they are saying is that big data was no longer an emerging technology. It had become normal and it was now part of everyone's life. Now first of all that's not quite the reality because a lot of businesses still struggle to make any good use of big data. And the other is, wouldn't we expect it then to move up the plateau of productivity, isn't that not exactly what the hype cycle is there for instead of just dropping a concept from the cycle.
Sandra: So the question there is - did big data exit the hype cycle and it was still a hype? Is it still a term that we use to refer to a nebulous collection of ideas and of initiatives and of practices and of technologies or did it exit around engineering or business maturity where it's actually something that we fully understand and it is now widespread and well understood of how we derive financial benefits from it.
Kai: I think it speaks more to the fact that big data had become this collection of things and had lost its precise meaning because all of a sudden everything that had to do with data, simple database applications, business intelligence, analytics that had existed for many many years were all of a sudden all called big data. So maybe they dropped it because it had lost any precise meaning. But I also want to point out that sometimes technologies just appear magically in the hype cycle like for example virtual reality appeared for the first time in the hype cycle in 2013 and it appeared right in the middle of the trough of disillusionment. No hype around it to proceed it, to move along and we all know that virtual reality used to be a thing much much earlier but completely disconnected from that it appeared in the trough and has moved slightly since then. It stood in the hype cycle and it's now sitting on the slope of enlightenment.
Sandra: Perched precariously on the slope of enlightenment.
Kai: But also there is now a lot of hype around virtual reality. I just came back from SIGGRAPH and there's the VR village. This is a thing. This is a hype now. So shouldn't it be on the mountain of the hype rather than on the slope of enlightenment, because a lot of what we see in VR at the moment is still very experimental. So some of those things do not make much sense to me.
Sandra: So what is it good for, is the question. If we are looking at the Gartner hype cycle - what is it good for? What can we do with it?
Kai: And does it describe reality or does it create reality? So because of the attention that the hype cycle gets there's reason to believe that whatever is on the hype cycle will actually become a thing and attract a lot of attention and therefore investment dollars that are flowing into those technologies.
Sandra: So I think it does provide a very compelling narrative structure. I think it provides us a way to talk about these technologies, to analyse where they are. I think there is a big danger there as well because as we mentioned in the beginning over 90 such hype cycles are created by Gartner because of course it depends what industry you're in, it depends what country you're in. It depends when you're looking at these things. So over 90 are created each year but only one gets reported. So this hype cycle is probably true in let's say North America at a particular point in time.
Kai: So the hype cycle is certainly done from some perspective and it is certainly very much embedded in our Western culture but it shapes the way in which we think about those technologies. But because it does so, I want to offer one observation which is that the downward curve and the upward slope behind it are much less populated than the rest of the curve. So what happened to big data is actually not that uncommon once things fall out of favour instead of tracking them along the curve they just disappear. Right. Which might have to do with the fact that we're all excited about what is hyped but we're not taking so much interest. No one likes their technology, what they are invested in, to be portrayed as being in the trough of disillusionment. So maybe this is just another sign of the fact that this hype cycle is about making and shaping reality rather than representing it.
Sandra: So it does offer a compelling narrative and possibly a way to actually shape the future. It does sometimes make useful observations. For instance this year that there is very heavy R&D spending from Amazon, Apple, Baidu, Google, IBM, Microsoft, Facebook there is this race for deep learning and machine learning patterns that will probably accelerate.
Kai: And all of these things are sitting right on top of the hype cycle, we have autonomous vehicles, machine learning, deep learning, virtual assistants, smart robots, all of these technologies are sitting right on the mountaintop. But I also want to offer the observation that on the upward slope we often see a lot of things that are not actually things yet like artificial general intelligence which are there.
Sandra: What is that?
Kai: That's just a pipe dream that was mostly dreamt by Elon Musk - the idea that artificial intelligence will acquire real intelligence and rise up and we've discussed this before. So I sometimes call this upward slope the bullshit slope because some of those ideas they never come to fruition they just slide back down into oblivion before they even become a thing.
Sandra: So whilst the hype cycle provides us with a compelling narrative and something to discuss what's in vogue now and to talk about whether artificial intelligence will become pervasive over the next few years we should be quite careful not to take it literally and not to think that all technologies go through this and that we are that good that either predicting the future or really identifying everything major that is coming up next or how soon they will mature.
Kai: So speaking of artificial intelligence this brings us to our second story which was sent in by our kean listener Greg.
Sandra: This is a story from the Guardian about an Irish woman who has failed the English oral test needed to stay in Australia and practise being a veterinarian.
Kai: So this story would end here if it wasn't for the fact that the test was actually done by an artificial intelligence based algorithm. Run by Pearson. Apparently there's a number of different organisations that offer English tests for prospective immigrants, people who want to obtain citizenship in Australia or obtain a visa. But Pearson is the only one who has the oral exam examined by computer or by an algorithm and not by a human examiner. This is also not the only time this has happened. In the wake of this becoming public, another Irish person has come forward and he has had the same experience. In both cases we're talking people who are highly educated, who are English language natives but where the algorithm apparently has problems understanding and making sense of their particular accent and both Sandra and I are now a little bit worried because...
Sandra: Speak for yourself I'm an Australian citizen.
Kai: So you just slipped through before this. So I'm in trouble here because I will have to sit this test to become a citizen and...
Sandra: If an Irish engineer can't pass it.
Kai: What chance do I have right? But seriously this raises questions again about what are we doing with those algorithms that create material effects on people's lives. Now both of these people yes they might have an accent but they are native English speaker so there should be no reason why these test scores come up with a low score.
Sandra: And of course these algorithms can get better and learn to recognise a wide variety of accents including weird ones like the ones we have but this is not the problem is it?
Kai: No and the reaction by Pearson is actually quite surprising who have staunchly defended their algorithm and said there is no problem there and these are the results. They are meant to be strict and the fact that these people couldn't pass the test has nothing to do with any flaws or inadequacies in the algorithms.
Sandra: So let's have another look at how these things work and where they're at work. So first how do these algorithms work?
Kai: So these artificial intelligence algorithms are a form of machine learning that learn to recognise patterns in data that they're trained with and they're usually quite good and we've talked about this before at recognising certain pattern in speech, in recognising patterns and images and all of these kind of things. But their quality very much depends on the training data and the extent to which they are trained to recognise variety in the data and we can only speculate but in this instance quite obviously the algorithm has problems making sense of a particular type of accent.
Sandra: So they're only as good as the data we manage to put in them and they're only as transparent as the companies who make them are willing to make them. Now the issue in this case was around immigration and there are people taking a language test and some of these people if they can afford it they can take a test with another company that will employ a human to do this. But what we want to highlight that these algorithms are also used in things like education or in issues around criminal justice or child welfare where the lack of transparency and the black box nature of these algorithms and also the type of data that's being used to train them become quite a significant issue.
Kai: Yes so the black box nature and the lack of transparency are really the biggest issues here and the fact that we are applying these algorithms in areas that have often material influence about people's lives such as whether someone is granted bail or they get a loan for their house. That's right. And so there was a recent article in Wired magazine titled "When government rules by software citizens are left in the dark". So this is from the US and it talks about cases where algorithms that are built by commercial entities are used in governments in the justice system to determine jail sentences but also whether someone is granted parole or bail. And the problem here is that it is very intransparent how those algorithms make those decisions what the factors are that flow into those decisions and what people can actually do to positively influence the outcomes of those decisions.
Sandra: So in this case the article brings up a very interesting research piece by a couple of law professors at George Washington University and at Rutgers University, an article that is coming out this month in the Yale Journal of Law and Technology that looks at algorithmic transparency and they started by looking at a specific case of someone who was paroled and then killed someone because there was a mistake in entering the data for the algorithm to then decide whether this person should or shouldn't get parole. And they found that there was a memorandum of understanding whereby the court was prevented from disclosing any information about the tool, about its development, about its operation, about its presentation. So what these researchers did was go back and within twenty three states request information about a number of tools used by governments to make algorithmic decisions in criminal justice and child welfare and education and so on. And they didn't get a lot for this. Many governments had said that they do not have the documents that they were being asked for about the programs that they are using, many agencies were not allowed to disclose them and so on.
Kai: It also became painfully clear that the people who are making decisions about using those algorithms really didn't know very much about how they worked and for them those algorithms were black boxes. The rationale behind using them is often an economic one. We're often talking government agencies that are heavily overloaded with work that just have to make do. Where an algorithm that helps making those decisions is just an absolute necessity in order to cope with the demands that are placed on those units and so they are often the last resort of keeping the system going. But it comes at the expense of the quality of the decision and the transparency in the process. And because of the inherent biases that inevitably creep into those algorithms, at the expense of fairness.
Sandra: So increasingly something that for a variety of disciplines and in this case it was law but clearly business is another one of those, increasingly questions about algorithmic ethics about what values we embed in these intelligent systems, how we train them and how we led them to decide at the expense of human decision making are things that need to be questioned and analysed and critically understood. There is not only a failure to regulate many of these things or to open the black box but also failure to recognise that there might be issues with its algorithms in the first place.
Kai: And we want to say this again and we've called this out before: the real danger of AI lies in the fact that we might apply it to problems where it's just not appropriate and that we trust decisions to AI that those algorithms are not really suited to making by themselves. And the main problem here is that when we employ these machine learning algorithms we're always making decisions about individual people on the basis of averages that become embodied in these algorithms by way of training them with training data.
Sandra: Given that we are unlikely to escape the world of algorithms, they are coming and we can talk about the variety of economic reasons and other reasons for which these will become pervasive, I think the issues that we must address are around understanding algorithmic ethics and understanding that having these algorithms does not absolve us of the challenge of changing, of removing bias from the world we live in, if we are going to change the data that we input into these algorithms.
Kai: Yes so we will never be able to create truly unbiased algorithms. What we must avoid is that those algorithms are the ultimate source of the decision that we make about individuals. We might use them as one source of information but the danger is that we trust those decisions to the algorithm because they come with the apparent clout of being rational and being correct and precise because it's the computer. But once we realise that what the computer does is work on inputs of the world we live in and making predictions on the basis of averages we might come to an appreciation of those algorithmic judgements as not absolute but just one more variable that a human decision maker can take into account in making decisions.
Sandra: And for that to happen we need to have meaningful transparency. So if a decision is made about me whether I get a credit or a loan or if I get shortlisted for a job I must be able to understand or see what were the parameters around which this decision was made and what was the process by which that decision was arrived at.
Kai: And by doing so we might eventually come to decide that in some parts of society especially when it comes to governments that have to apply fairness to its citizens the application of those algorithms might actually not be appropriate at all.
Sandra: Our final story for today comes from Rolling Stone magazine and it looks up how Spotify playlists can create hits so basically looks at the inner workings of Spotify. So last month Spotify reported it has about 60 million subscribers. That's about a 100 percent jump from last year.
Kai: For comparison its closest competitor Apple Music has just 27 million subscribers.
Sandra: And more than half of these Spotify users listen to the services playlists - their top playlists have as much as 16 million subscribers and for artists it seems it has become the thing to do - get on one of the Spotify playlists. We want to look at how this is changing the structure of the music industry and the way in which artists interact with their audiences.
Kai: So playlists play a big role on Spotify. So on the one hand Spotify itself curates a number of these playlists but they also have famous artists and individual users who can create these and share them with other users. And some of those playlists have become really really popular. So for individual artists with their songs it's incredibly important that they become listed and played on these playlists and the article speaks about how it can really make and break the careers of young and upcoming artists, whether they are being listed on or fail to be included in any of those playlists on Spotify.
Sandra: So radio has always been about the power interplay between the tastes of the people who were putting the songs on the radios and the number of listens. This time however with Spotify that has become an unambiguous number - you can actually track exactly how many play or how many listens those songs get. So once they get on it it becomes a more objective game. But getting on it is the big step. However there are a number of things that Spotify and services like it have changed for the music industry. First is they got rid all together of albums, so the way in which music gets released, when it gets released, how it gets released has changed altogether. The artful part of making music was also about combining a certain number of songs together to tell a larger narrative.
Kai: And it was always up to the artist to make that curation and come up with the story you tell across different songs of an album.
Sandra: That has been done away all together with streaming services. The same thing is now happening actually two genres in music. We used to try to play rock or blues or R&B or hip hop. It is now more of what is the activity that you're doing. There is the mood, there is the time of the day, there is the running playlist, the driving playlist, the barbecue playlist. So more and more of these streaming services in order to maximise the...
Kai:...the exposure of songs and also the rotation and the streaming.
Sandra: They need to know exactly what you're doing while you're doing this to better tailor the content.
Kai: But streaming services have also changed the economics in the music industry. The money that artists make of streaming of their songs through these playlists is infinitesimally small when compared to the sales that you'd generate from an album. So realistically very few artists can actually live off the income they generate from streaming services. But on the other hand these playlists and the streaming services provide the platform for becoming well known and have the exposure to fans on the back of which artists can then organise their own headline tours and make money off the concerts that they give. So it's a way of exposure and becoming popular. It's not so much an income source in itself.
Sandra: Speaking of the economics of it, it also allows companies like Spotify to actually derive huge benefits from this. One of the examples brought up in the Rolling Stone article is the fact that elevator music pays big.
Audio: Elevator music playing
Sandra: The elevator music genre racks up tens of millions of streams which means that these would potentially be fairly big paydays for the copyright owners. Now it's not clear and the article makes this point who that copyright owner is because it seems that Spotify might itself be commissioning a lot of this music and they would be the owners of that. So in a way becoming something akin to Netflix, once you start figuring out what people like you could basically tailor the content and start building that content yourself.
Kai: So there was another article in The Guardian which reports that Spotify denies filling popular playlists with fake artists. So the accusation being made there, and Spotify is not very forthcoming and transparent about this, is that Spotify is commissioning this music and then it's released through fake artists or under pseudonyms to its platform included in those playlists that people use as elevator music background to the activities that they're doing and that they are in fact making money off of these playlists that flows directly or via a middleman into their own pockets therefore changing the model of being just in an intermediary or a platform. But now becoming an original content producer much like Netflix.
Sandra: There are a number of other questions that we can raise not only about the economics of the music industry but also about music itself. So one of the things that's been argued is that music has become a much more passive experience given these playlists. While many of us used to curate our own feeds much like we used to curate news by buying certain newspapers or certain magazines or by recording certain things on TV, this playlist experience has made music a much more passive experience also much more utilitarian experience. We listen to stuff while we're running or while we're exercising and much less of an art form.
Kai: And further changes are introduced like how and where we are consuming music, for example Spotify is now accessible through systems such as Amazon's Alexa or Google Home where we can just ask Spotify to play a particular song and given the platform nature and the gatekeeper all that Spotify now place and the game they have created around listing songs and creating playlists, a new phenomenon has crept up which is the creation of cover songs that are being released under names that are just a little bit different to the original artist's songs fooling systems such as Alexa and Google Home into playing the wrong version of the original song. Therefore siphoning off some of those dreaming cents into the pockets of artists who are busily creating those cover songs. And there's been an article in The Verge a couple of years ago that speaks to this phenomenon.
Sandra: So this still seems to be a phenomenon undergoing much transformation. I don't think we're at the end of a process but rather at the very beginning figuring out what this would transform to. If we go back to algorithms and machine learning and so on there is a chance that these playlists will someday become quite personalised to our own taste where we could feed back to the system what we like what we don't like. We see that in the case of streaming services like Pandora who have just exited Australia.
Kai: Now speaking of music here is:
Audio: "Robot of the Week"
Sandra: You've just listened to 1069 dancing robots breaking the world record for most robots dancing simultaneously.
Kai: Listed in the Guinness Book of Records now.
Sandra: A technology company in China has broken this record with these synchronised robots about 18 inches tall dancing together. What do we think about this?
Kai: My question is: why oh why? None of these robots had their own brain. They were centrally orchestrated and I really don't get it. We marvel at synchronised dancing when a hundred dancers move in perfect unison at parades or the Olympics or dance events right so this is magnificent when people train and they have the skills to do this but robots?
Sandra: But we created them in our image.
Kai: Yeah we find it spectacular when humans move like robots but robots we expect them to move like robots. So we're now able to centrally control one thousand and sixty nine robots to all move alike? We would expect this to happen. So what's so brilliant about this? I don't get it.
Sandra: But it looks so cute.
Kai: And creepy.
Sandra: And that's all we have time for this week.
Kai: Thank you for listening.
Sandra: Thanks for listening.
Outro: This was The Future, This Week made awesome by the Sydney Business Insights team and members of the Digital Disruption Research Group. And every week right here with us our Sound Editor Megan Wedge who makes us sound good and keeps us honest. You can subscribe to this podcast on iTunes, Soundcloud, Stitcher, or wherever you get your podcasts. You can follow us online, on Flipboard, Twitter or sbi.sydney.edu.au. If you have any news you want us to discuss please send them to sbi@sydney.edu.au.
Sandra: Is the ringing in my ear or in the room?
Kai: It's in your head.
Sandra: You're kidding?
Kai: I don't hear any ringing. You want to take the call?
Close transcript