Sydney Business Insights and Daniel Kahneman
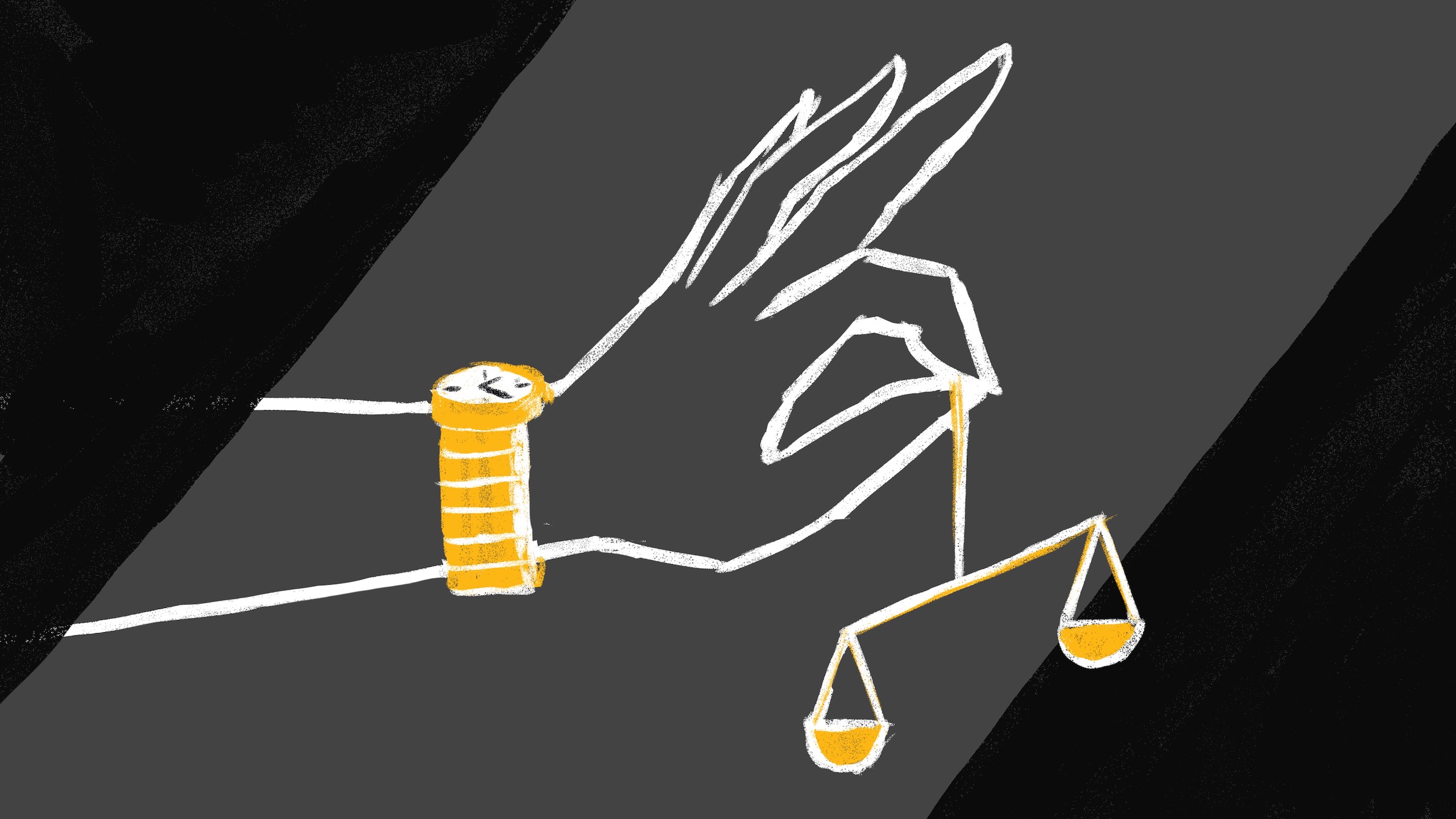
Thinking with Daniel Kahneman
Should we let algorithms make decisions for us?
Nobel prize winner and renowned author, Professor Daniel Kahneman, believes that the best way to eliminate noise when making decisions is to eliminate judgement altogether.
Join Sandra Peter as she talks with Professor Kahneman, author of Thinking, Fast and Slow and winner of the 2002 Nobel Memorial Prize in Economic Sciences.
Shownotes
Professor Kahneman’s recount of his personal and professional story
Professor Kahneman’s Princeton University profile and CV
Publications
Thinking, Fast and Slow, Daniel Kahneman’s best-selling 2011 book
International Differences in Well-Being, a joint book on understanding and comparing well-being across countries and cultures
Heuristics and Biases: The psychology of intuitive judgment, a compilation of the most influential research in the heuristics and biases
Choices, values and frames, Daniel Kahneman and Amos Tversky discuss choice in risky and riskless contexts
Well-being: The foundations of hedonic psychology, an account of current scientific efforts to understand human pleasure and pain, contentment, and despair
Judgment Under Uncertainty: heuristics and biases, an insight into judgements and how to improve them
Attention and Effort, a summary of a decade of research on attention and the role of perception
Dan Lovallo and Daniel Kahneman’s article on how optimism undermines executives’ decisions in the Harvard Business Review
How to overcome the high, hidden cost of inconsistent decision making in the Harvard Business Review
Other links
Daniel Kahneman talking about his friendship with Amos Tversky
The Undoing Project: A Friendship That Changed Our Minds, Michael Lewis’ book on the professional and personal relationship between Danny Kahneman and Amos Tersky
Professor Kahneman’s 2010 TED Talk on the riddle of experience versus memory and his TED interview with Chris Anderson
Graphic summary of Thinking, Fast and Slow
Thinking, Fast and Slow – Talks at Google
Follow the show on Apple Podcasts, Spotify, Overcast, Google Podcasts, Pocket Casts or wherever you get your podcasts. You can follow Sydney Business Insights on Flipboard, LinkedIn, Twitter and WeChat to keep updated with our latest insights.
Sydney Business Insights is a University of Sydney Business School initiative aiming to provide the business community and public, including our students, alumni and partners with a deeper understanding of major issues and trends around the future of business.
Daniel Kahneman is Professor of Psychology and Public Affairs Emeritus at the Princeton School of Public and International Affairs and was awarded the 2002 Nobel Prize in Economic Sciences.
Share
We believe in open and honest access to knowledge. We use a Creative Commons Attribution NoDerivatives licence for our articles and podcasts, so you can republish them for free, online or in print.
Transcript
This transcript is the product of an artificial intelligence - human collaboration. Any mistakes are the human's fault. (Just saying. Accurately yours, AI)
Intro From the University of Sydney Business School, this is Sydney Business Insights, the podcast that explores the future of business.
Sandra When the amazing Daniel Kahneman, the 85 year old Nobel prize winning psychologist, has agreed to grant you an interview, the last thing you want is a problem with the phone connection. Good morning, Professor Kahneman.
Daniel Hello, how do you do? So you solved your technical problem?
Sandra So here's a quick heads up that my interview with Danny Kahneman showcases both his superb mind, but alas, also some less than superb sound. But we weren't going to let the opportunity slip away. It's not every day that you get to hear from a Nobel prize winning intellectual and bestselling author.
Sandra Thank you so much for talking with me today. How are you?
Daniel I'm just fine. Then there was the matter of the Kahneman naming protocol...
Daniel Oh, my title, I mean you know, I, I don't use my titles, but, you know, if you want to call me Professor Daniel Kahneman. But I don't usually call myself Professor Kahneman.
Sandra Would you prefer I call you something else?
Daniel I mean, everybody calls me Danny, I'm not very formal, but I'm, you know, I'm glad to do whatever is convenient. You want me to call myself Professor Kahneman, I'll do that gladly.
Sandra Just professor of psychology.
Daniel Yeah, sure. And Daniel Kahneman, Professor of Psychology and Public Affairs Emeritus at Princeton University.
Sandra I have to start with Professor Kahneman's reaction to the surprising public enthusiasm for his best-selling book Thinking, Fast and Slow. I say surprising, because this is a profoundly intellectual book. It is, after all, Professor Kahneman's research into how the human brain thinks and makes decisions. Professor Kahneman conducted much of his research with his friend and colleague, the mathematical psychologist Amos Tversky. Tversky tragically died of cancer at just 59 years. Professor Kahneman dedicated his 2002 Nobel Prize for economics to Tversky, humbly stating that he considered it a joint prize.
Sandra Having conversations with my colleagues over the past few weeks, everybody is very excited we get to talk to you, and pretty much every single person I've talked to has read your book, Thinking, Fast and Slow. It's been an international best-seller for years now. What do you think has made it such a popular success?
Daniel Well, I think it appeals to something that is introspectively obvious that everybody can recognise, those two ways in which ideas come to mind. The faster way and the slower way, and everything else is secondary I think, people recognise themselves in that idea. But the ironic aspects of this is that the two system idea is really not my idea, I just made it popular and I have given it my own twist, but took the idea and even the terminology from other people.
Sandra So how did you make it your own?
Daniel Well, I spent a lot of time thinking about it, and the first hundred pages of the book, which is I think more than most people read, but they develop a point of view on the two systems. And I think one of the aspects of this that are the most helpful is the language of systems. Which is ironic again, because in psychology you are really not supposed to explain the mind or behaviour, by the behaviour of little people inside your head. And yet, this is precisely what I do in Thinking, Fast and Slow. I have System 1 and System 2. And I picked that in improper language very deliberately, because it makes it very easy for people to think in terms of agents. Thinking in terms of agents is a lot easier than thinking in terms of categories. Agents have properties, they have characters, they have propensities, and you attribute traits to them very, very easily. And that is, I think, part of the success of the book.
Sandra At the time you started researching this and you start the writing about this, there were different prevailing views of what human nature is and what thinking is like. In economics for instance, there was a very different view of how we are as people, and how we think.
Daniel Yes. There was very little by way of a field of study of thinking under uncertainty, there were really maybe half a dozen psychological papers. There was a fairly rich economic literature, and the literature on the foundation of the statistics, which is really about the logic of thinking under uncertainty, and the logic of decision-making under uncertainty. And then there was a bridging move that economists adopted, which was to take the logical theory and use it as a descriptive theory of how people actually behave. And that was a very good thing to have around, because we could use it as a target. I mean it's obviously a theory that people are completely rational and completely logical, and follow the axioms of utility theory and the actions of probability theory. And that story is so obviously false, that is quite easy to find flaws in it, or to prove it wrong in amusing ways.
Sandra So how did you come to that realisation? Because, you know, we're in a business school here and everybody talks about making data-driven decision, and you always say that no one's ever made that decision because of a number, they needed a story. There are so many ways in which human decision-making is wrong. How did you come to that realisation?
Daniel Most of the research that Amos Tversky and I did, actually happened when we were just the two of us walking around and talking to each other. Because we would set problems for each other, and to ourselves, and what was amusing to us was to set problems where we had a clear intuition, and we also knew that that intuition was wrong, because we knew the normatively appropriate theory. So that was how we came to realisation that people are imperfect, it's because our own intuitions were imperfect. I was first acquainted with that very early in my life, when I was in my early 20s and serving in the Israeli army as a psychologist. And I encountered there incorrect intuitions, both in my own thinking and in the thinking of other people. So I can give you an example, I was interviewing candidates for Officer School, and I noticed that sometimes when interviewing someone, I had the distinct impression that I knew them. That I had sort of an insight into their true nature. But then, I also knew enough from the statistics on our inability to predict any criterion, that my impressions of knowing the person, that was an illusion, I was just generating it myself. So I coined the phrase the illusion of validity, actually when I was 22 years old and serving in the Israeli army. It became a technical term some 15 or 20 years later. But that's where my interest in this began.
Sandra So how does the illusion of validity come about?
Daniel The illusion of validity comes about basically when you have a clear interpretation of the situation which is internally coherent, and you do not really see any alternative ways of understanding the situation. So we tend to be very confident when no alternatives to our interpretations come to mind. And this really happens a lot, because part of perception, and part of impression formation is that once you begin to form an impression, it tends to suppress alternative interpretations of the same evidence. So we are designed to come up with a single interpretation. This is really the case with vision, and much of our thinking about intuition came from of thinking about vision. And in vision you see things one way, or another way. You don't see things two ways at the same time, or very rarely.
Sandra How can we overcome this?
Daniel Well, by slowing down. And you can't overcome it in general. I mean, you know, we, we think the way we think, and we can't slow ourselves down all the time. When the stakes are high, and in the context of a decision with consequences, and typically in the context of decisions on the job, and decisions that are made with a group, we call them singular decisions, the important decisions. Then, you can do a little better. Then, you can make yourself slow down. Then, you can critique your own thinking. Then, you can arrange for different parts of the committee to play different roles. There are several techniques for overcoming intuition. And as I now think about it, for delaying intuition, until all the facts are in.
Sandra Do you think there is a good role for intuition to play in high-stakes environments? You spoke about work in the Israeli army.
Daniel I think it's essential for people to be confident in their decisions, especially when they get to the phase of implementation. So when you are deciding, you can be in doubt. But at a certain point you decide, and then you have to march on. And at that point there is really not much alternative for intuition, to get a sense of certainty under complex situations. But it's also very important not to reach it prematurely, because many of our intuitions come too early, and many of our intuitions are wrong.
Sandra So is the old saying of counting to 10 and then doing what you're about to do?
Daniel The general advice when you want to overcome problems with what I call 'fast thinking' is to engage in 'slow thinking'. And to do that systematically, and carefully and slowly.
Sandra What do you think are some really good examples of the application of your work? And I'm thinking organisations, or government or other implications.
Daniel I think, for example, one of the starting points in the work that I'm doing now, and the books that I'm writing now, is that people overuse the notion of bias. But actually there are other kinds of error, and in particular, the one that we're writing about. There is noise, just unreliability, it's not bias. It's a very different thing, which produces errors. Now, people have been quite influenced, and not only by us, but the word bias is in the culture, and there are lists of biases in the culture. You look at Wikipedia, it has 200 cognitive biases. So lots of people have, in one way or another, tried to overcome biases. How successful they have been, I have no idea. You know, we have not suggested direct applications. There are a few things where I do know, oh here's an example. One of the ideas we developed was the idea of the outside view. That is that when you're facing a problem, instead of focussing on the problem itself, you should try to view that problem as an instance of a broader category of problems. And then you should look at the statistics that you can come up with about the outcomes in similar cases, to predict what will happen in this particular case. And there are now other people leading the charge on this, namely in particular a professor at Oxford named Flyvbjerg. But I think there is a requirement, or a recommendation, in the American Planning Association to use the outside view whenever a forecasting exercise is required.
Sandra You talked a bit about noise and I understand you are writing in that space. Can you talk to me a little bit about the importance of noise, and what we mean by it, and where does it occur?
Daniel Well, when you look at the judgments that people make, and they start in organisations. So if you look at underwriters looking at the same risk. Now, normally the organisation has a lot of underwriters, and there are many people who can be called on to deal with any particular case that comes by. And typically, one underwriter will take care of it. Now, what we found in doing research on this is that this is a major lottery, that is who the underwriter is that will take the case can make a very large difference to... in effect the organisation will decide because these underwriters have the authority to speak for the organisation. And we know that they vary a lot. And that variation is what we call system noise. And there is noise in the judicial system. There are huge differences between judges in the American judicial system in the sentence they will set for exactly the same criminal committing the same crime. So that's noise. And wherever we look, whether there is judgment actually, there is noise. Because judgment is an informal integration of information, and because it is informal different people are going to do it in different ways. And indeed, we use the term 'it's a matter of judgment' when we expect differences. But the reason we're writing a book about this is that the differences are much, much larger than people typically think. So in the insurance company that I worked with, they were completely unaware that they had a noise problem. But in fact, they had a major noise problem. And the same is true in many other organisations. So that's noise, it's just the unreliability of different agents for the organisation, producing different responses, or being likely to produce different responses to the same problem. There is also noise in individual decisions, in singular decisions, in the sense that there are many ways in which a decision or a judgment that looks inevitable to the person making it, could in fact have been made quite differently. And it's quite often fairly arbitrary which decision happens to be made. So that's true for both important decisions and more routine decisions. There's a lot of noise. It's a good topic for a book. We think.
Sandra It's a fantastic topic for a book, looking forward to reading it. So are there ways in which we can mitigate the effects of noise? I'm guessing we can't eliminate it from our lives, but there might be ways in which we can at least be aware of it.
Daniel Yes, so the best way to eliminate noise is to eliminate judgment. So by using algorithms instead of judgment. And the algorithms have been successful for a long time, and a very simple algorithm, I'm not even talking of, of AI where you have nonlinear systems that perform very well. But simple linear combinations of variables do better than people in most judgments. There've been hundreds of studies comparing judgements to simple rules for combining information. And the simple rules beat people about 50 percent of the time, and the remaining 50 percent tends to be a tie. So no question, the best way of getting rid of errors of judgment is to get rid of judgment if you can. Now, if you must use judgment, then you must structure it. Break up the problem, stay very fact-based, and assessing what we call 'mediating assessments', break up the problem into facets. Actually, you know, this goes back to my work in the Israeli army, 63 years ago. I set up an interviewing system in the Israeli army, which I think might very well still be in use, anyway five or ten years ago it was still in use, pretty much unchanged from the way I'd set it up. And it was an interviewing method. And the idea of that interviewing method was that instead of trying to form an impression of how good a soldier, the interviewee, was going to be. What you did, you assessed six separate traits by asking factual questions about the current behaviour, and the current outcomes of the individual, and collecting facts and making separate and independent judgments on separate attributes. And at the end of that process, it turns out when people make an intuitive judgment, after producing six separate judgments, their intuitive judgment is much better than it would have been if they had tried to go directly to intuition. So that's what I was saying earlier when I said tried to delay intuition, because you'll get a better intuition if you delay it. So this is a noise mitigation system, actually. And it turns out we know that structured interviews are more reliable, and more valid, than unstructured interviews. And so we have a line when we think of important decisions, we say that options in important decisions are pretty much like candidates in a personnel selection situation. And the same ideas that work for evaluating candidates are applicable in many cases to evaluating options in decision-making. So that's the tack that we're taking on noise mitigation in judgement.
Sandra I want to go a bit further into how you view the role of AI and of algorithms and of machine learning in this. And you spoke about linear versus non-linear systems. Do you think there is risk in building in some of the biases, for lack of a better word, that we already have in the data that we feed into these algorithms?
Daniel I think there is real deep confusion about what we mean by bias in this context, because what there is, I don't think that it is true that we feed our predictive biases into the algorithms. Now, there is bias in life. There are differences between groups, you know, there are differences in how well they do, in what they accomplish. That's just a fact. And now the differences might come about because of discrimination, say. If there are ethnic differences in achievements, that could well be because of discrimination. But if your criterion is, say, whether people will be promoted or not. If that's the criterion, because this is the way that success is evaluated, then the algorithm will predict that criterion. And if the criterion is biased, the algorithm will be biased. But the bias is in life. It's in the criterion, it is not that we are feeding our predicted biases into the device. The learning device learns not by asking us what we think, but by trying to predict an outcome in the environment. And now what will typically happen is if the world is biased, that is, if say there is a difference between in some achievement, then I predict the system will be biased in the sense that it will favour the group that is the more successful group, and disfavour the others. And that is not bias, it's just an inevitable side effect of trying to predict the criterion that is possibly biased or anyway that discriminates between groups. So I think algorithms are getting a bad rap, is my impression.
Sandra I would agree with you that they are. For instance, you talked about judges making decisions, and so on. We know, for instance, some of that data comes from over-policing certain areas. So of course, you will have higher incidence of crime in areas that you over-police, rather than other areas. And it's quite difficult to figure out just how much of that is already built into the ways we see the world.
Daniel This is a particularly interesting case, because it's true that if you're measuring arrests, then there will be movement of arrests in areas that are over-policed. But then if you ask why are they over-policed? Then it might be that there was more crime there. So there is something that could be self-reinforcing, and if you're measuring by arrests, then clearly you are using not only the problem, but the solution to the problem as part of the criterion, and that can be problematic. But it's a good example, actually, where some bias, I don't know if it's a bias. Where imagine the following, that an appropriate response to high crime is to over-police, that is, to disproportionately police. Then you'll find out that you'll make a lot more arrests when you over-police, including for minor crimes that you wouldn't bother with in other areas. And so there'll would be discrimination that way.
Sandra What's the equivalent of a recourse to a System 2 in the case of algorithms?
Daniel We cannot evaluate systems by isolated cases. The only proper way to evaluate a System is statistically. And what is the case, is that our attitude, the public attitude to mistakes that are made by people and to mistakes that are made by algorithms. That attitude is quite different. And you know, we'll be much more shocked, we are much more shocked, when a self-driving car kills a person, than when a person driving a car kills a person. We really hate the idea of algorithms making mistakes that have important consequences in the lives of real people. So there is that emotional reaction that complicates things.
Sandra Where do you see most promise for AI in the future, or for machine learning or for algorithms? What areas do you see most promise in?
Daniel Well, it's developing at such a speed that it's fairly clear that prediction is involved, and there is a good criterion to predict, and there is a mass of data, then machine learning is it. That's the way to do it. It's clearly superior to intuition. It is clearly superior to standard statistics. And it's just a matter of getting it enough material and you'll get spectacular results. Now, there are many domains of life, and many domains of thinking and judgment and decision-making, where AI is not a factor yet. So, artificial intelligence does not do causal thinking, Artificial intelligence that does not yet do scenario thinking. So there are many areas in which artificial intelligence just is not playing yet, but in the areas in which it can play, machine learning is simply superior to anything that it competes with.
Sandra Still some ways to go, but promise then.
Daniel Well, I don't know if it's a promise or a threat, because the impact of highly intelligent systems on human life is going to be quite problematic. The thing that I find fascinating is the replacement of professional judgment by algorithms. This is happening in medicine. It's going to happen in the law. I mean, it's clear that the medical assistant is going to be a better diagnostician than the physician that it's assisting, that's not going to take much time. This is already beginning to happen, and it's going to be the same in many other areas. And I even have a fantasy of it being the case that machine learning might have better business intuitions than people. So that decision-making at the high level, deciding whether or not to merge, or whether or not to acquire a company, those fateful decisions that sometimes are taken. I wouldn't be surprised if within two decades or so there will be programs that do it better than most CEOs.
Sandra Do you see any risk in that?
Daniel The main risk I see is that CEOs will resist the implementation of these programs. This is really in part what happened to decision theory. Fifty years ago formal decision theory, I know that Amos Tversky and I thought that it was going to conquer the world, and many other people thought that decision analysis was going to conquer the world. In fact, there is very little decision analysis being practiced today. And I think the reason is that leaders hated to be second-guessed by the decision analysts. You just don't want the problems that you solved by the magic of your intuition and experience, to be solved by a technician applying a rule, applying an algorithm. That's going to create interesting problems.
Sandra But then what becomes of the role of the leader or the CEO? You talked a bit in the beginning about how we need to tell stories, and people believe and follow stories, or follow narratives. These decisions will be made solely-based on data, and there will be a number at the end of it, you know it's that way because it's 7. What happens then to the role of leaders? Or what becomes of them?
Daniel That's what I see as the threat. I won't venture to predict anything about social responses and how things will play out. We know that this type of forecasting is effectively impossible. Technical forecasting is possible, so that we have a pretty good idea by now the range of problems to which machine learning is applicable. And so we can predict that wherever it's applicable and wherever there are enough data, that will happen. But what the social reaction to it will be, and how people will make their peace with it, if they do, that now I wouldn't venture to predict, I have no idea. I'm very surprised by the confidence with which some people predict the future, because there is ample evidence to show that the future is very hard to predict.
Sandra Do you think we have only good tools for thinking about the future? What do you use as tools to think about the future?
Daniel I use modesty. Actually, I don't, I make a lot of intuitive predictions and they're mostly wrong, but my thinking hasn't been modified by the research that I have done. But we know about this miserable record of long-term forecasting in science, in technology, and certainly in political and strategic events. So we know that people are able to forecast certain developments, like AI's spreading. They're able to forecast short term developments, and there is all that work that Philip Tetlock is doing, and Barbara Mellers, on superforecasting, which is very interesting, but it's limited to the short-term. Medium and long-term, there are people who claim that they have had major successes, but I'm a sceptic. We can't see around corners. We can see up to the next corner, but that's not very far.
Sandra Let me take you to one other area that you have made a significant impact on. And I want to go towards more the hedonic psychology side, and life satisfaction versus happiness. And you've more recently talked quite a bit about how it is more important for people to be satisfied, to experience life satisfaction rather than to be, you know, merely happy. Could you share your understanding of satisfaction as distinct from happiness, and why you rank satisfaction as more important?
Daniel Yeah. Well, actually I don't. So let me elaborate on that. The distinction is fairly clear. I mean, happiness as I define it is happiness in the moment, but every moment there is a particular hedonic level of experience. You're in a good state or in a bad state, or somewhere in between. And you can take the average of that, or the integral over time. And that's the measure of happiness. And life satisfaction is a completely different sort of animal. I mean, it's how you feel about your life when you think about your life. But most of the time we don't think about our life, most of the time we just live. So those are very different concepts of well-being and they can be measured separately. And it turns out that what you would do to maximize your happiness, and what you would do to maximize your life satisfaction, are quite different. Now you maximize life satisfaction by looking for pretty conventional measures. You know, you're satisfied if you're conventionally successful. Happiness is largely to do with people, being with people you love and being loved by people. So there's a real contrast between those two. Now, I used to have strong opinions about this, and my strong opinion was that happiness is the real thing, and that life satisfaction is just something that people think about their life. But after a few years of defending that point of view, I realized that I was painting myself into a corner, that I was proposing a theory of well-being, which is not what most people are trying to achieve, because mostly people are trying to achieve life satisfaction. They're not trying to be happy. And so it's not that I think that they should look for life satisfaction, I have no view on this. Well, I mean, I have conflicting views on this, but it's clear that what people are motivated by is primarily the stories they can tell about their life, and that's more likely satisfaction. So, that's... What I have been saying recently on this is that I think that the focus on achieving happiness is exaggerated, and that the proper focus of society should be reducing misery. And that what you would do as a society to maximize happiness and to reduce misery, is really not the same thing. And, and that I believe one is more important than the other. But I'm not having much influence.
Sandra What do you think is the most important thing we could do to reduce misery, either from a public policy point of view or an individual point of view?
Daniel Well, thinking in terms of public policy, you want to know where there is suffering, and you want to alleviate it. Now, probably most of the suffering at any one time is being done by a few people. I mean, we had an estimate, about 10 percent of people do about 90 percent of the suffering. I can elaborate if you're curious that I made that strange claim, but..
Sandra Yes, please.
Daniel Well, you can look at any one time, and you have the reports of how happy people were say at, at one o'clock, and you're looking at a thousand people. And then you look at two o'clock, and at three o'clock and so on, and you can count whether there is suffering, or there is no suffering. And then it turns out that of the total amount of people who would check that they suffer over time, that 90 percent of those checks would be done by about 10 percent of people. So suffering is really very evenly distributed in society. And so there are some categories of suffering that are inevitable, like people grieving for a true disaster that's happened. But a lot of suffering is mental illness. So if you want to reduce suffering, the major focus should be mental illness. Here I'm less sure of myself, but there have been claims that loneliness is a big problem for some categories of people, especially widowers I think, and then that would be a problem that you would want to address.
Sandra Well in the US, it's been declared an epidemic by the surgeon general, bigger than the obesity epidemic.
Daniel Yeah. And there is suffering that is caused by social problems. And there is a lot of suffering that is caused by poverty. So I would say that the data that we have indicates that in terms of emotional happiness, getting richer or getting higher income doesn't really add a lot to your emotional happiness. But what is true, is that if you are poor, you're suffering. You're suffering and miserable, emotionally as well as having poor life satisfaction. So thinking about poverty and about the safety net, those are would-be implications of the focus of misery reduction.
Sandra How can we find better ways of talking about misery? We tend to talk about happiness and happiness research, rather than misery, just like we talk about equality and empowerment rather than inequality research. Yet in health we managed to talk about obesity and heart disease, not about centres for fit, healthy people.
Daniel Yeah, there is a bias in the language. We talk of length and not of shortness. We talk of depth, and not of shallowness. There is that bias in the language with one of the directions in those dimensions, and happiness is a marked one. And unhappiness is the lack of happiness. So I think that's a genuine obstacle. But, if people train their mind on measuring misery, they will develop tools that are not the same tools that are used to measure life satisfaction. And if that's your focus, then the first requirement is to measure it. And anything that you don't measure is not going to have enough impact on policy. But things that you measure have a good chance of affecting policy.
Sandra What have you been lucky with?
Daniel Professionally, I've been most lucky. But, you know, working Amos Tversky was clearly the turning point in my life and it made me what I am professionally. And that was really luck. The luck was that we got along very well and enjoyed each other’s company. And we happened to complement each other, so that we found each other at the right time in our careers, and we spent 10 or 12 years, you know, working as one, I would say superior mind, as a very, very good mind indeed when the two of us were working together. That's luck. Now, you know, we did something with it. But even where we did, there were elements of luck. Many of the things that were the most successful, they were not planned. They happened, and they happened because of a series of accidents.
Sandra One last difficult question is how do you think about failure in life? Are there any good tools to think about failure? I'm in a business school, and it seems all we talk about is success, and how to be more successful, or how to build on success, how to achieve it. But we really seldom talk about failure. Does your research, your body of work, and your life experience have lessons about failure?
Daniel Actually, the work I've done all my life has been about errors, not about success. So I am a specialist in sort of short-term and small failures, this is really my specialty. Now, big failures, painful failures, it's interesting, I haven't thought about that in the same way that I've thought about success being lucky. I mean, clearly there's a lot of bad luck that causes failures, but there is also a lot of stupidity that causes failures, and a lot of bad character that causes failures. So, it should be I suppose, the mirror image of success. That is, there are many opportunities to go wrong. And if you use too many of those opportunities, you're not going to be very successful. And, you know, that's the luck, that's an interaction between luck and character. You surprise me actually by your question, because some of my friends, notably Gary Klein, who is a psychologist, believes in experts and believes in intuition, and he really doesn't like the way I think, except that we are friends. Now, he would accuse me of being very negative, of being focussed on failure avoidance. And there are quite a few people writing about business. Gary is one of them, I think Phil Rosenzweig is another who object to the line of work that I've been associated with, because of its exaggerated focus on failure avoidance, rather than on seeking success. So I'm not sure I recognise the situation that you you're describing. Clearly we talk a lot more about success because people want to be successful, and they believe that they can be told how to do it, which is an illusion.
Sandra You mentioned that you've had little failures along the way, and I'm guessing this is experiments...
Daniel No, I mentioned that I had disasters, that was mostly, you know, in my personal life. But I don't think I mentioned many failures. But failures are routine in academic work and in thinking work. I'm not sure whether I'm in a minority, I think I am, but I actually enjoy changing my mind, for example. And the occasion for changing your mind is always when you find that you've been wrong. And for me, this is a real joy, finding that I've been wrong, because that discovery means that I've learned something. So failure and success are inextricably linked, in my experience of thinking, and discovering that you've been wrong, of correcting yourself. And it's those failures, those challenges, that make the kind of work I do exciting.
Sandra I do want to thank you for really your generosity with your time today, and the insights that you've brought to so many people. You've really made a difference.
Daniel Well thank you, that's very kind and I enjoyed our conversation.
Sandra Links to the books, articles and talks mentioned in this podcast are available in the shownotes. This podcast was recorded and edited by Megan Wedge, and researched and produced by Jacquelyn Hole.
Outro You've been listening to Sydney Business Insights, the University of Sydney Business School podcast about the future of business. You can subscribe to our podcast on iTunes, Stitcher, Libsyn, Spotify, SoundCloud or wherever you get your podcasts. And you can visit us at sbi.sydney.edu.au and hear our entire podcast archive, read articles, and watch video content that explore the future of business.
Close transcript